Power analysis in statistics is an essential tool for designing studies that yield accurate and reliable results, guiding researchers in determining optimal sample sizes and effect sizes. This article explores the significance of power analysis in statistics, its applications, and how it supports ethical and effective research practices.
Power analysis in statistics refers to the process of determining the likelihood that a study will detect an effect or difference when one truly exists. In other words, power analysis helps researchers ascertain the sample size needed to achieve reliable results based on a specified effect size, significance level, and statistical power.
By grasping the concept of power analysis, researchers can significantly enhance the quality and impact of their statistical studies.
Unlocking the Essentials of Power Analysis in Statistics
The basics of power analysis in statistics revolve around understanding how sample size, effect size, and statistical power interact to ensure meaningful and accurate results. Understanding the basics of power analysis involves familiarizing yourself with its key concepts, components, and applications. Here’s an overview of these fundamentals:
1. Key Concepts
- Statistical Power: This refers to the probability that a statistical test will correctly reject the null hypothesis when it is false. In practical terms, it measures a study’s ability to detect an effect if one exists. Power is usually set at a threshold of 0.80 (80%), meaning there is an 80% chance of correctly identifying a true effect.
- Effect Size: Effect size quantifies the strength or magnitude of the effect being studied. It helps determine how large of an effect is expected, which influences the required sample size. Common measures include:
- Cohen’s d: Used for comparing means between two groups.
- Pearson’s r: Quantifies both the strength and direction of the linear relationship between two variables.
- Alpha Level (Significance Level): This is the probability of making a Type I error, which occurs when a researcher incorrectly rejects a true null hypothesis. The alpha level is typically set at 0.05, indicating a 5% risk of concluding that an effect exists when it does not.
- Sample Size: This refers to the number of participants or observations in a study. Generally, a larger sample size increases the statistical power, enhancing the likelihood of detecting a true effect.
2. Types of Power Analysis
- A Priori Power Analysis: Conducted before data collection, this type helps determine the necessary sample size to achieve the desired power for a specific study design.
- Post Hoc Power Analysis: Conducted after the data is collected, this analysis evaluates the power of the study based on the observed effect size and sample size. While it can provide insights, it is often criticized for its limited usefulness.
- Sensitivity Analysis: This examines how changes in parameters (like effect size, alpha level, or desired power) affect the required sample size, providing a better understanding of the robustness of the study design.
3. Applications of Power Analysis in Effective Study Design
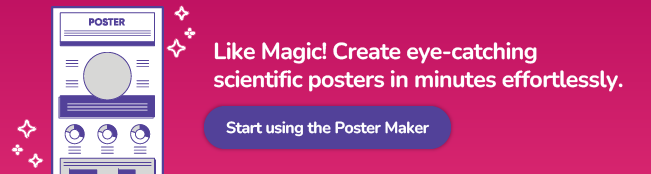
- Study Design: Power analysis is crucial during the planning stages of research to ensure an adequate sample size is determined for robust results.
- Grant Proposals: Funding agencies may require power analysis to justify the proposed sample size, demonstrating the study’s validity and potential impact.
- Ethical Considerations: Conducting power analysis helps prevent underpowered studies, which can lead to Type II errors (false negatives) and may waste resources or expose participants to unnecessary risks.
Components of Power Analysis
Power analysis involves several critical components that influence the design and interpretation of statistical studies. Understanding these components is essential for researchers aiming to ensure that their studies are adequately powered to detect meaningful effects. Here are the key components of power analysis:
1. Effect Size
- Definition: Effect size quantifies the magnitude of the difference or relationship being studied. It is a critical factor in determining how large a sample size needs to be to detect a true effect.
- Types:
- Cohen’s d: Measures the standardized difference between two means (e.g., the difference in test scores between two groups).
- Pearson’s r: Measures the strength and direction of the linear relationship between two variables.
- Odds Ratio: Used in case-control studies to measure the odds of an event occurring in one group compared to another.
- Importance: A larger effect size typically requires a smaller sample size to achieve the same power level, while a smaller effect size necessitates a larger sample to detect the effect.
2. Sample Size
- Definition: Sample size refers to the number of participants or observations included in the study. It directly influences the power of the statistical test.
- Calculation: Determining the appropriate sample size involves considering the desired effect size, significance level, and desired power. Statistical formulas or software tools can assist in these calculations.
- Impact: A larger sample size increases the likelihood of detecting a true effect, reduces variability, and leads to more precise estimates of population parameters.
3. Significance Level (Alpha)
- Definition: The significance level, commonly denoted as alpha (α), is the threshold for determining whether a statistical result is statistically significant. It indicates the likelihood of committing a Type I error, which involves rejecting a true null hypothesis.
- Common Values: The most frequently used significance level is 0.05, indicating a 5% risk of concluding that an effect exists when it does not.
- Role in Power Analysis: A lower alpha level (e.g., 0.01) makes it harder to achieve statistical significance, which may require a larger sample size to maintain the desired power.
4. Power (1 – Beta)
- Definition: Statistical power is the probability of correctly rejecting the null hypothesis when it is false, effectively detecting an effect that truly exists. It is calculated as 1 minus the probability of making a Type II error (beta, β).
- Common Standards: A power level of 0.80 (80%) is commonly accepted, indicating an 80% chance of detecting a true effect if it exists. Researchers may choose higher power levels (e.g., 0.90) for greater assurance.
- Influence: Power is influenced by effect size, sample size, and significance level. Increasing the sample size or the effect size will enhance the power of the study.
Why Power Analysis Matters
Power analysis in statistics is vital for ensuring sufficient sample size, enhancing statistical validity, and supporting ethical research practices. Here are several reasons why power analysis is important:
1. Ensures Sufficient Sample Size
- Avoids Underpowered Studies: Conducting a power analysis helps researchers determine the appropriate sample size needed to detect a true effect. Underpowered studies (those with insufficient sample size) are at risk of failing to identify meaningful effects, leading to inconclusive results.
- Reduces Wasted Resources: By calculating the necessary sample size upfront, researchers can avoid recruiting more participants than needed, thus conserving time and resources while still ensuring valid results.
2. Enhances Statistical Validity
- Improves Accuracy of Findings: Power analysis helps ensure that studies are designed to yield reliable and valid results. Adequate power increases the likelihood of correctly rejecting the null hypothesis when it is false, thus enhancing the overall quality of the research findings.
- Supports Generalizability: Studies with sufficient power are more likely to produce findings that can be generalized to a broader population, increasing the impact and applicability of the research.
3. Guides Research Design Choices
- Informs Study Planning: Power analysis helps researchers make informed decisions regarding study design, including the selection of appropriate statistical tests and methodologies. This planning is critical for maximizing the effectiveness of the research.
- Considers Practical Constraints: Researchers can weigh the desired power against practical constraints such as time, budget, and participant availability. This balance is essential for conducting feasible and meaningful studies.
4. Facilitates Ethical Research Practices
- Protects Participant Welfare: Conducting power analysis ensures that studies are appropriately powered, which helps protect participants from being involved in studies that lack sufficient rigor. Underpowered studies may expose participants to unnecessary risks without providing valuable insights.
- Promotes Accountability: Researchers who utilize power analysis demonstrate a commitment to methodological rigor and ethical standards, fostering a culture of accountability in scientific research.
5. Supports Grant Applications and Publication Standards
- Strengthens Grant Proposals: Funding agencies often require power analysis as part of grant applications to justify the proposed sample size and demonstrate the study’s potential impact and validity.
- Aligns with Publication Guidelines: Many academic journals and conferences expect researchers to provide power analyses as part of the methodology section, reinforcing the importance of this practice in scholarly communication.
6. Enhances Interpretation of Results
- Informs the Context of Findings: Understanding the power of a study can help researchers interpret their results more effectively. If a study fails to detect an effect, researchers can assess whether the lack of findings is due to insufficient power rather than the absence of an actual effect.
- Guides Future Research: The insights gained from power analysis can inform future studies, helping researchers design more robust experiments and refine their hypotheses.
Avoiding Type II Errors
Power analysis is essential not only for detecting true effects but also for minimizing the risk of Type II errors in statistical research. Understanding Type II errors, their consequences, and the role of power analysis in avoiding them is crucial for researchers.
Definition of Type II Error
- Type II Error (β): A Type II error occurs when a statistical test fails to reject the null hypothesis when it is actually false. In simpler terms, it means that the study fails to detect an effect that is present. The symbol β represents the probability of committing a Type II error.
- Illustration: For example, if a clinical trial is conducted to test a new medication’s effectiveness, a Type II error would occur if the trial concludes that the medication does not work (fails to reject the null hypothesis) when, in fact, it is effective.
Consequences of Low Power
Low power in a statistical study significantly increases the risk of committing Type II errors, which can lead to various consequences, including:
- Missed Opportunities for Discovery
- Underestimating True Effects: When studies are underpowered, they are less likely to detect true effects, leading to the erroneous conclusion that no effect exists. This may result in missed opportunities for scientific advancement, particularly in fields where detecting small effects is crucial, such as medicine and psychology.
- Wasted Resources
- Inefficient Use of Funding: Underpowered studies can lead to wasted time, funding, and resources. If a study fails to detect an effect due to low power, additional studies may be required, further straining resources without generating useful insights.
- Misleading Conclusions
- False Sense of Certainty: A failure to reject the null hypothesis due to low power may lead researchers to draw misleading conclusions about the absence of an effect. This can propagate misconceptions in the literature and skew future research directions.
- Compromised Research Integrity
- Erosion of Credibility: A series of underpowered studies yielding non-significant results can undermine the credibility of the research area. When researchers consistently fail to detect effects, it raises questions about the validity of their methodologies and findings.
- Impediments to Clinical Practice
- Impact on Treatment and Policy Decisions: In applied fields such as medicine and public health, Type II errors can have real-world consequences. If a treatment is ineffective but believed to be effective due to the absence of significant findings in underpowered studies, patients may receive suboptimal care.
- Ethical Concerns
- Participant Exposure: Conducting studies with low power can expose participants to risks or interventions without the potential for meaningful contributions to scientific knowledge. This raises ethical concerns about the justification of the research.
Balancing Resources with Power Analysis in Research
Designing an efficient study is critical for obtaining valid results while maximizing resource utilization and adhering to ethical standards. This involves balancing available resources and addressing ethical considerations throughout the research process. Here are key aspects to consider when aiming for an efficient study design:
1. Balancing Resources
- Resource Assessment: Begin by assessing the available resources, including time, funding, personnel, and equipment. Understanding these constraints helps researchers make informed decisions about study design, sample size, and methodology.
- Optimal Sample Size: Use power analysis to determine the optimal sample size that balances the need for statistical power with the available resources. A well-calculated sample size minimizes waste while ensuring that the study has enough power to detect meaningful effects.
- Cost-Effective Methodologies: Explore cost-effective research methodologies, such as online surveys or observational studies, that can yield valuable data without extensive financial investments. Utilizing technology and data analysis tools can also streamline processes and reduce costs.
- Collaboration: Collaborating with other researchers, institutions, or organizations can enhance resource sharing and provide access to additional funding, expertise, and data. This can lead to more comprehensive studies that still respect resource limitations.
- Pilot Studies: Conducting pilot studies can help identify potential issues in the study design before the full-scale research is implemented. These preliminary studies allow for adjustments that can enhance efficiency and effectiveness.
2. Ethical Considerations
- Informed Consent: Ensure that all participants provide informed consent before participating in the study. This means clearly communicating the study’s purpose, procedures, potential risks, and benefits, allowing participants to make informed decisions about their involvement.
- Minimizing Harm: Design studies to minimize potential risks and harm to participants. Researchers must weigh the potential benefits of the research against any possible adverse effects, ensuring that the well-being of participants is prioritized.
- Confidentiality and Data Protection: Implement robust measures to protect the confidentiality of participant data. Researchers should anonymize data where possible and ensure that sensitive information is securely stored and accessed only by authorized personnel.
- Review by Ethical Committees: Before conducting the study, obtain approval from relevant ethical review boards or committees. These bodies evaluate the study design for ethical considerations, ensuring compliance with established standards and guidelines.
- Transparent Reporting: Commit to transparent reporting of study results, including both significant and non-significant findings. This fosters trust within the research community and supports the advancement of knowledge by preventing publication bias.
- Inclusivity in Research: Strive for inclusivity in study design, ensuring that diverse populations are represented. This not only enriches the research findings but also aligns with ethical considerations of fairness and justice in research practices.
Steps to Conduct Power Analysis in Statistics
Conducting a power analysis is essential for designing statistically robust studies. Below are the systematic steps to conduct power analysis effectively.
Step 1: Define Your Hypothesis
- State Null and Alternative Hypotheses:
- Clearly articulate your null hypothesis (H₀) and alternative hypothesis (H₁). The null hypothesis typically states that there is no effect or difference, while the alternative hypothesis proposes that there is an effect or difference.
- Example:
- Null Hypothesis (H₀): There is no difference in test scores between two teaching methods.
- Alternative Hypothesis (H₁): There is a difference in test scores between two teaching methods.
- Determine Expected Effect Size:
- The effect size is a measure of the magnitude of the phenomenon of interest. It can be defined as small, medium, or large, depending on the context and the research field.
- Common measures of effect size include Cohen’s d for comparing two means and Pearson’s r for correlation.
- Estimating the expected effect size can be based on previous studies, pilot studies, or theoretical considerations. A larger expected effect size generally requires a smaller sample size to achieve adequate power.
Step 2: Choose Significance Level
- Typical Alpha Values:
- The significance level (α) is the probability of committing a Type I error (rejecting the null hypothesis when it is true). Common alpha values are 0.05, 0.01, and 0.10.
- An alpha of 0.05 indicates a 5% risk of concluding that a difference exists when there is no actual difference.
- Impact of Stringent Alpha Levels:
- Choosing a more stringent alpha level (e.g., 0.01) reduces the likelihood of a Type I error but increases the risk of a Type II error (failing to detect a true effect). It may also require a larger sample size to maintain adequate power.
- Researchers must carefully consider the trade-off between Type I and Type II errors when selecting the alpha level based on the specific context of their study.
Step 3: Estimate Sample Size
- Role of Sample Size in Power:
- Sample size directly impacts the power of a statistical test, which is the probability of correctly rejecting the null hypothesis when it is false (1 – β). Larger sample sizes increase the power of the study, making it more likely to detect an effect if one exists.
- Typical power levels sought in research are 0.80 (80%) or higher, indicating a 20% chance of making a Type II error.
- Tools and Software for Calculation:
- Various tools and software packages can assist researchers in conducting power analysis and estimating sample sizes, including:
- G*Power: A free tool widely used for power analysis in different statistical tests.
- R: The pwr package in R provides functions for power analysis.
- Statistical software: Many statistical software packages (e.g., SPSS, SAS, and Stata) include built-in functions for conducting power analysis.
- Various tools and software packages can assist researchers in conducting power analysis and estimating sample sizes, including:
Your Creations, Ready within Minutes
Mind the Graph platform is a powerful tool for scientists looking to enhance their visual communication. With its user-friendly interface, customizable features, collaborative capabilities, and educational resources, Mind the Graph streamlines the creation of high-quality visual content. By leveraging this platform, researchers can focus on what truly matters—advancing knowledge and sharing their discoveries with the world.
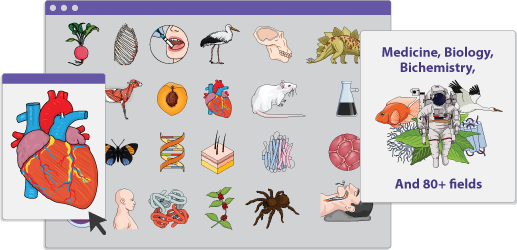
Subscribe to our newsletter
Exclusive high quality content about effective visual
communication in science.