Nullhypotesens signifikans er et grunnleggende konsept innen statistisk testing, og hjelper forskere med å avgjøre om dataene deres støtter en bestemt påstand eller observasjon. Denne artikkelen tar for seg begrepet nullhypotesesignifikans, hvordan det brukes i forskning, og hvor viktig det er for å ta datadrevne beslutninger.
In its simplest form, the null hypothesis suggests that there is no significant effect or relationship between the variables you’re testing. In other words, it assumes that any differences you observe in the data are due to random chance, not because of a real effect.
The importance of the null hypothesis lies in its objectivity. But, let’s stop with this, as feeding too much at the start will confuse you. Let us learn about the nullhypotesens signifikans fra bunnen av!
Forstå nullhypotesens betydning i forskning
The null hypothesis is central to understanding null hypothesis significance, as it represents the assumption of no effect or relationship between variables in statistical testing. In other words, it suggests that whatever you’re testing—whether it’s a new medication, teaching method, or any other intervention—has no impact compared to the standard or baseline scenario.
The purpose of a null hypothesis is to provide a starting point for analysis, where you assume there’s no change or difference.
You can think of the null hypothesis as a default position that you’re trying to disprove or reject. Instead of directly assuming that your experiment will have an effect, you first consider that nothing has changed.
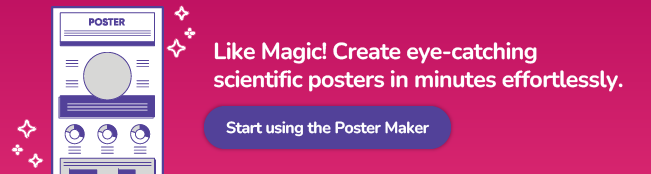
This helps you approach the situation objectively and prevents you from jumping to conclusions without evidence. By starting with the assumption of “no effect,” you can rigorously test your idea using data, and only if the evidence is strong enough can you reject the null hypothesis and claim that something significant has occurred.
Rollen i vitenskapelige eksperimenter
Nullhypotesen spiller en avgjørende rolle i den vitenskapelige forskningsprosessen. Den skaper et tydelig rammeverk for eksperimentering og dataanalyse. Når du gjennomfører et eksperiment, er målet ditt vanligvis å finne ut om en bestemt variabel påvirker en annen.
Du kan for eksempel ønske å finne ut om et nytt legemiddel reduserer symptomer mer effektivt enn placebo. Nullhypotesen i dette tilfellet vil si at legemiddelet ikke har bedre effekt enn placebo, og oppgaven din er å samle inn data som enten støtter eller utfordrer denne ideen.
By establishing a null hypothesis, you also introduce the concept of “falsifiability” into your experiment. Falsifiability means that your hypothesis can be tested and potentially proven wrong. This is important because it ensures your scientific claims are based on measurable data, not assumptions or guesses.
Eksempler på nullhypoteser
Eksempel 1: Testing av en ny diettplan
Imagine you’re testing a new diet plan to see if it helps people lose weight compared to a regular diet. Your null hypothesis would be: “The new diet has no effect on weight loss compared to the regular diet.” This means you’re starting with the assumption that the new diet doesn’t work any better than what people are already eating.
Once you have this null hypothesis, you can collect data by having two groups of people—one following the new diet and the other following their regular diet. After analyzing the data, if you find that the group on the new diet lost significantly more weight than the control group, you might reject the null hypothesis. This would suggest that the new diet plan does have a positive effect.
Eksempel 2: Undersøkelse av søvnens innvirkning på testresultater
In another scenario, you might want to study whether more sleep improves students’ test scores. Your null hypothesis would be: “There is no relationship between the amount of sleep and students’ test scores.” In other words, you assume that how much sleep students get doesn’t affect their performance on tests.
You would then collect data on students’ sleep habits and their test scores. If you find that students who get more sleep consistently score higher, you might reject the null hypothesis and conclude that more sleep does indeed improve academic performance.
However, if your data shows no meaningful difference between well-rested students and those who sleep less, you would fail to reject the null hypothesis, meaning there’s no evidence to suggest that sleep has a significant impact on test results.
I begge eksemplene fungerer nullhypotesen som et grunnlag for testingen og hjelper deg med å vurdere om dataene du samler inn, gir nok bevis til å trekke meningsfulle konklusjoner.
Relatert artikkel: Definer en hypotese: Det første trinnet i en vitenskapelig undersøkelse
Betydningen av nullhypotesens signifikans ved testing
Formålet med nullhypotesen
Konseptet nullhypotesens signifikans underbygger forskning ved å gi et nøytralt utgangspunkt for å evaluere vitenskapelige påstander på en objektiv måte. Formålet er å gi et nøytralt utgangspunkt som hjelper deg å teste om resultatene av eksperimentet skyldes tilfeldigheter eller en reell effekt.
When you perform research, you often have a theory or prediction in mind—something you hope to prove. The null hypothesis, however, assumes that there is no effect or relationship. For example, if you’re testing whether a new drug improves patient recovery, the null hypothesis would state that the drug has no effect compared to a placebo.
Denne antakelsen er avgjørende fordi den holder analysen objektiv. Ved å ta utgangspunkt i at ingenting har endret seg eller blitt bedre, sikrer du at alle konklusjoner du trekker, er basert på solide bevis, og ikke på personlige oppfatninger eller forventninger.
Det hjelper deg med å opprettholde en objektiv tilnærming, slik at du unngår å trekke forhastede konklusjoner bare fordi du ønsker at hypotesen din skal være sann.
Additionally, the null hypothesis provides a standard against which you can measure your findings. Without it, you wouldn’t have a clear baseline to compare your results, making it difficult to know if the data actually supports your theory.
I alle eksperimenter fungerer nullhypotesen som en sikkerhet for at konklusjonene dine er underbygget av data, ikke antakelser.
Rolle i hypotesetesting
Hypotesetesting dreier seg om nullhypotesens signifikans, det vil si å vurdere om observerte resultater er signifikante eller bare skyldes tilfeldig variasjon. Det er her nullhypotesen blir sentral. Du starter med å sette opp to hypoteser: nullhypotesen (som antar at det ikke finnes noen effekt) og alternativhypotesen (som antyder at det finnes en effekt eller en sammenheng).
Hypotesetesting innebærer vanligvis at man samler inn data og analyserer dem for å se hvilken hypotese dataene støtter. Først antar du at nullhypotesen er sann. Deretter gjennomfører du eksperimentet og samler inn data for å teste denne antakelsen.
Deretter bruker du statistiske metoder for å analysere dataene, for eksempel ved å beregne p-verdier eller konfidensintervaller. Disse metodene hjelper deg med å vurdere sannsynligheten for at de observerte resultatene skyldes tilfeldigheter.
Hvis dataene viser at det er svært usannsynlig at de observerte resultatene inntreffer under nullhypotesen (vanligvis bestemt av en p-verdi som er lavere enn en viss terskel, for eksempel 0,05), forkaster du nullhypotesen.
This doesn’t necessarily mean that the alternative hypothesis is absolutely true, but it suggests that there is enough evidence to support it over the null hypothesis.
On the other hand, if the data doesn’t provide strong enough evidence to reject the null hypothesis, you “fail to reject” it. This means you don’t have enough proof to claim a significant effect or relationship, so the null hypothesis remains valid.
Testing the null hypothesis is essential because it allows you to make informed decisions about the significance of your results. It helps you avoid false positives, where you might incorrectly conclude that a relationship exists when it doesn’t.
Faktorer som påvirker nullhypotesetesting
The significance level, often represented by the symbol α (alpha), is a key factor in hypothesis testing. It is the threshold you set to determine whether the results of your experiment are statistically significant, meaning whether the observed effect is likely real or simply due to chance.
Vanligvis velger man et signifikansnivå på 0,05 (eller 5%). Det betyr at man er villig til å akseptere en 5% sjanse for at resultatene skyldes tilfeldig variasjon i stedet for en sann effekt.
Think of the significance level as a cut-off point. If the p-value, which measures the probability of observing the effect if the null hypothesis is true, is smaller than the significance level, you reject the null hypothesis. This suggests that there is enough evidence to conclude that a real effect or relationship exists. On the other hand, if the p-value is larger than the significance level, you fail to reject the null hypothesis, indicating that the data doesn’t provide strong enough evidence to support a significant finding.
Signifikansnivået du velger, påvirker hvor streng du er i testingen. Et lavere signifikansnivå (f.eks. 0,01 eller 1%) betyr at du er mer forsiktig med å forkaste nullhypotesen, men det reduserer også sannsynligheten for å finne signifikante resultater.
Et høyere signifikansnivå (f.eks. 0,10 eller 10%) øker sjansene for å finne signifikante resultater, men gjør det også mer sannsynlig at du feilaktig forkaster nullhypotesen. Derfor er valget av signifikansnivå viktig, og det bør gjenspeile konteksten for studien din.
Type I- og type II-feil
Ved hypotesetesting kan det oppstå to typer feil: Type I- og type II-feil. Disse feilene er direkte knyttet til utfallet av testen og valget av signifikansnivå.
Type I-feil
A Type I error occurs when you reject the null hypothesis even though it is actually true. In other words, you conclude that there is an effect or relationship when there really isn’t one.
This is also known as a “false positive” because you are detecting something that isn’t actually there.
The significance level you set (α) represents the probability of making a Type I error. For example, if your significance level is 0.05, there is a 5% chance that you might incorrectly reject the null hypothesis when it’s true.
The implications of a Type I error can be serious, especially in fields like medicine or pharmaceuticals. If a new drug is tested and a Type I error occurs, researchers might believe the drug is effective when it isn’t, potentially leading to harmful consequences.
To reduce the risk of a Type I error, you can choose a lower significance level. However, being too cautious by lowering the significance level too much can also have drawbacks, as it may make it harder to detect real effects (which leads to another type of error—Type II error).
Type II-feil
A Type II error occurs when you fail to reject the null hypothesis when it is actually false. In simple terms, this means you are missing a real effect or relationship that does exist. This is known as a “false negative” because you are failing to detect something that is actually there.
The probability of making a Type II error is represented by the symbol β (beta). Unlike the significance level, which you set before testing, β is influenced by factors such as the sample size, the effect size, and the significance level.
Større utvalg reduserer sjansen for type II-feil fordi de gir mer data, noe som gjør det lettere å oppdage reelle effekter. På samme måte er større effektstørrelser (sterkere sammenhenger) lettere å oppdage og reduserer sannsynligheten for å gjøre en type II-feil.
Type II-feil kan være like problematiske som type I-feil, spesielt når det står mye på spill.
Hvis du for eksempel tester om en ny medisinsk behandling virker, og du gjør en type II-feil, kan du konkludere med at behandlingen ikke har noen effekt når den faktisk har det, og dermed forhindre at pasienter får en potensielt gunstig behandling.
Det er viktig å balansere risikoen for begge typer feil. Hvis du fokuserer for mye på å unngå type I-feil ved å sette et svært lavt signifikansnivå, øker du risikoen for type II-feil, det vil si at du går glipp av reelle funn. På den annen side, hvis du prøver å unngå type II-feil ved å sette et høyere signifikansnivå, øker du sjansen for å gjøre en type I-feil. Derfor er det avgjørende med nøye planlegging og vurdering av studiens kontekst.
Les også: Hypotesetesting: Prinsipper og metoder
Praktiske anvendelser av nullhypotesens signifikans
Eksempler fra hverdagen
The concept of a null hypothesis isn’t just limited to complex scientific studies—it actually applies to many scenarios in everyday life. To help you understand it better, let’s look at two simple, relatable examples where the null hypothesis is used.
Eksempel 1: Testing av et nytt treningsopplegg
Imagine you’ve come across a new workout plan that claims it will help you lose more weight compared to your current routine. The null hypothesis here would be that the new workout plan doesn’t make a significant difference in your weight loss compared to your existing routine. In other words, you’re starting with the assumption that the new plan won’t help you lose more weight.
You could then test this by following both workout plans over a set period, tracking your weight loss with each one. If, after collecting enough data, you find that you’re losing significantly more weight with the new plan, you might reject the null hypothesis, concluding that the new plan is effective.
On the other hand, if your weight loss results are similar, you’d fail to reject the null hypothesis, meaning the new plan didn’t provide any additional benefit.
Example 2: Evaluating a Sleep App’s Effectiveness
Let’s say you download a sleep app that claims it will help improve your sleep quality. You want to test whether using this app actually leads to better sleep. Your null hypothesis here would be that the app has no effect on your sleep quality.
To test this, you could track your sleep patterns for a week without using the app and then for another week while using it. If you find that your sleep improved significantly after using the app—such as falling asleep faster or waking up less frequently—you could reject the null hypothesis. This would suggest that the app really did improve your sleep. But if the data shows no noticeable difference, you’d fail to reject the null hypothesis, meaning the app likely doesn’t have any measurable effect.
Vanlige misoppfatninger om nullhypotesens signifikans
Det kan være utfordrende å tolke nullhypotesens signifikans på grunn av vanlige misoppfatninger, for eksempel at statistisk signifikans er det samme som praktisk betydning.
Vanlige misoppfatninger
One common misconception is that if you fail to reject the null hypothesis, it means the null hypothesis is definitely true. This isn’t the case. Failing to reject the null hypothesis simply means you don’t have enough evidence to support the alternative hypothesis.
It doesn’t prove that the null hypothesis is correct, but rather that the data you collected doesn’t provide enough support for a different conclusion.
Another misunderstanding is believing that rejecting the null hypothesis means your findings are automatically important or valuable. Statistical significance only means that the observed effect is unlikely to have occurred by chance, based on the data you’ve collected. It doesn’t necessarily mean the effect is large or practically meaningful.
Du kan for eksempel finne et statistisk signifikant resultat som viser en ørliten effekt som har liten innvirkning i den virkelige verden.
Unngå fallgruver
To avoid these pitfalls, it’s essential to remember that statistical significance is just one piece of the puzzle. You should also consider practical significance, which asks whether the effect you’ve observed is large enough to matter in the real world.
Selv om en ny undervisningsmetode fører til en liten forbedring i testresultatene, er det ikke sikkert at den er stor nok til å rettferdiggjøre en endring av hele læreplanen.
Another important piece of advice is to make sure you’re not relying on p-values alone. P-values can help you decide whether to reject or fail to reject the null hypothesis, but they don’t tell you the full story.
It’s also crucial to look at the size of the effect and the confidence intervals around your results. These give you a clearer picture of how reliable your findings are.
Lastly, avoid the temptation to manipulate your data or keep testing until you find a significant result. This practice, known as “p-hacking,” can lead to false conclusions. Instead, plan your study carefully, collect enough data, and follow through with a proper analysis to ensure your conclusions are based on solid evidence.
In summary, while null hypothesis testing can be a powerful tool, it’s important to interpret the results carefully and avoid common misconceptions. By focusing not just on statistical significance but also on the real-world relevance of your findings, you’ll make more informed and meaningful decisions based on your data.
Nullhypotesen er et grunnleggende element i statistisk testing, og gir et objektivt utgangspunkt for å analysere hvorvidt observerte effekter er reelle eller skyldes tilfeldigheter. Ved å være nøye med å fastsette et signifikansnivå kan du balansere risikoen for type I- og type II-feil, noe som sikrer mer pålitelige resultater.
Ved å anvende nullhypotesen på hverdagsscenarioer får du hjelp til å se dens praktiske verdi, samtidig som du unngår vanlige misforståelser og fokuserer på både statistisk og praktisk signifikans for å sikre at konklusjonene dine er meningsfylte.
Når du forstår disse konseptene, kan du ta datadrevne beslutninger med større trygghet.
Les også: Hvordan skrive en hypotese
Stor gjennomslagskraft og større synlighet for arbeidet ditt
Det er viktig å forstå nullhypotesens signifikans, men det kan være avgjørende å kommunisere funnene dine på en effektiv måte. Mind the Graph gir forskere verktøy for å lage visuelt engasjerende infografikk og diagrammer som gjør komplekse statistiske begreper lettere å forstå. Plattformen vår hjelper deg med å dele innsikten din med klarhet og gjennomslagskraft, enten det gjelder akademiske presentasjoner, forskningsartikler eller offentlig formidling. Begynn å forvandle dataene dine til visuelle bilder i dag.
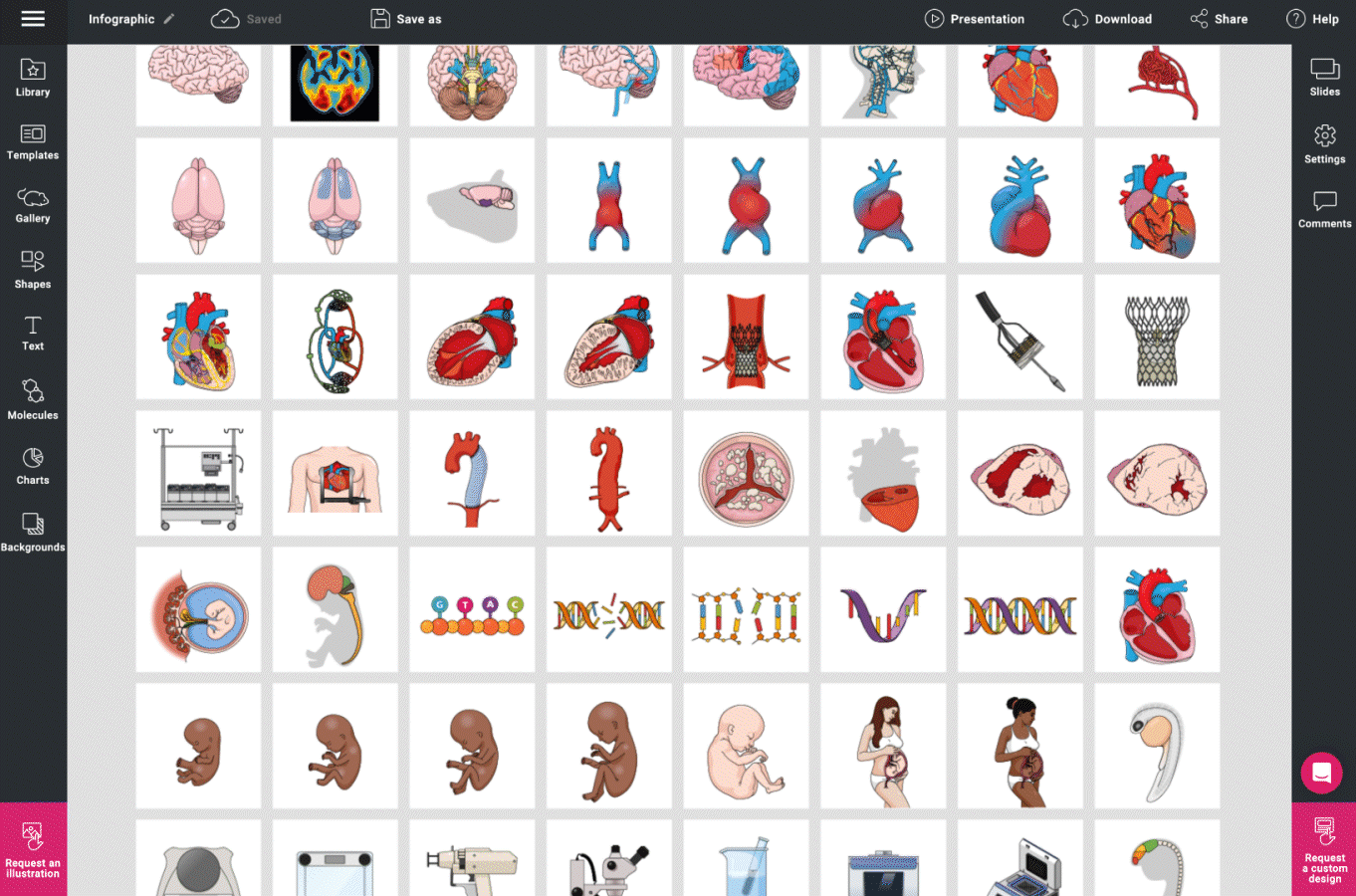
Abonner på nyhetsbrevet vårt
Eksklusivt innhold av høy kvalitet om effektiv visuell
kommunikasjon innen vitenskap.