Have you ever been struck with curiosity about how researchers draw concrete conclusions from groups of data that, at first glance, seem as mysterious as an ancient code? Well, it becomes a little less enigmatic once you understand the magic behind post hoc testing in the context of ANOVA – Analysis of Variance. This statistical method isn’t just a tool; it’s akin to Sherlock Holmes’ magnifying glass used for uncovering hidden truths within myriad numbers. Whether you are a student grappling with your thesis data or a seasoned researcher aiming for robust results, unlocking the power of post hoc tests can elevate your findings from interesting to groundbreaking.
Understanding ANOVA and Post Hoc Testing
When delving into the intertwining concepts of ANOVA and post hoc testing, think of them as partners in the quest for accurate analysis. They empower us to peer beyond average values and explore deeper nuances between multiple group comparisons – but let’s proceed step by step.
Related article: Post Hoc Analysis: Process and Types of Tests
Introduction to ANOVA and its purpose in statistical analysis
Analysis of Variance, or ANOVA as it is commonly known among statisticians, stands tall as one of the most powerful tools in their arsenal. It serves a critical function – distinguishing whether there are any statistically significant differences among group means in an experiment involving three or more groups. By comparing variances within individual groups against variances between these groups, ANOVA helps reject or retain the null hypothesis that no variance exists other than through random chance.
Explanation of post hoc testing and its importance in ANOVA
While identifying significance across large sets is essential, what happens when ANOVA tells us “something” differs but does not specify “what” and “where”? Cue post hoc testing! Short for “after this”, post hoc testing follows up on the trail left by ANOVA’s omnibus test. Its mission? To pinpoint exactly which pairs or combinations amongst our groups exhibit significant differences thus enabling researchers to make informed decisions with impeccable precision.
Overview of the process of post hoc testing in ANOVA
Engaging with post hoc testing always comes after obtaining a significant result from an ANOVA omnibus test — hence its retrospective name. Picture this process consisting largely of:
- Selecting the appropriate post hoc test: Depending on design specifics and error-rate tolerance.
- Adjusting p-values: Correcting for inflated risks associated with making multiple comparisons.
- Interpreting results within context: Ensuring practical significance aligns with statistical findings.
This disciplined approach safeguards against spurious conclusions while extracting valuable insights lying dormant within datasets. Armed with this advanced yet accessible understanding can set anyone on a path toward mastery over their data narratives.
ANOVA Omnibus Test
Analyzing data sets with more than two means to understand if at least one differs from the others is where an Analysis of Variance (ANOVA) becomes essential. But before we dive into the intricacies of post hoc testing in ANOVA, it’s crucial to grasp the foundational assessment—the ANOVA omnibus test. Think of it as a detective story where the initial evidence points towards the possibility of a suspect but doesn’t pinpoint exactly who.
Related article: One-Way ANOVA: Understanding, Conducting, and Presenting
Detailed explanation of the ANOVA omnibus test
The ANOVA omnibus test stands out because it allows us to compare multiple group means simultaneously rather than conducting numerous tests for each significance level of every possible pair, which would undoubtedly escalate type I error risks—the false-positive rate. The “omnibus” in its name suggests that this test takes an overall perspective—it’s checking collectively whether there’s any statistically significant difference among group means.
Here’s how it unfolds: We start by calculating separate variances within groups and among groups. If our groups are quite uniform internally but differ vastly from each other, that’s a solid indicator that not all group averages are equal. Essentially, we’re looking for between group b within-group variability that can’t be explained by chance alone relative to within-group variability—what we’d expect from random fluctuations.
Understanding the F-statistic and its interpretation
When performing an ANOVA omnibus test, we compute what is called the F-statistic—a value derived from dividing between-group variance by within-group variance. A large F-value may indicate significant differences among group means because it suggests that between-group variability is higher compared to within-group variability.
But here’s where caution is paramount: The F-statistic follows a specific distribution under the null hypothesis (which posits no difference among our group means). Before leaping to conclusions based on just this statistic, we reference this F-distribution considering our degrees of freedom related to both between-groups and within-groups, giving us a p-value.
Interpreting the results of the omnibus test
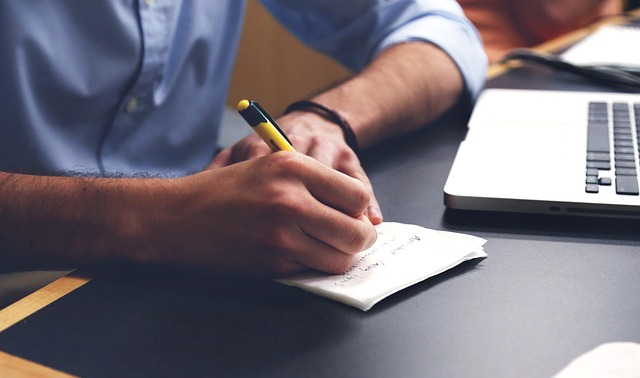
So you’ve run your analysis and have your hands on that all-important p-value after comparing your calculated F-statistic against the appropriate distribution—but what now? If this p-value dips below your threshold level—often 0.05—we reach rejection territory for our null hypothesis. This suggests strong evidence against no effect across all groups.
However—and this part is crucial—an overarching rejection does not guide us about which particular means differ nor by how much; it doesn’t specify ‘who done it’ in our earlier detective analogy. It merely informs us that there is something worth investigating further in our line-up—which leads us directly onto post hoc testing in ANOVA—to unravel these detailed disparities between specific pairs or combinations of groups.
Understanding when and why post hoc tests follow an ANOVA omnibus test ensures researchers handle their findings responsibly without jumping prematurely or incorrectly to associations or causal statements—all while aiding clear communication in their fields of study.
Need for Post Hoc Testing in ANOVA
Exploring the limitations of the omnibus test
When I dissect the complexity of statistical analysis, it’s essential to acknowledge that while tools like Analysis of Variance (ANOVA) are powerful, they have their boundaries. The ANOVA omnibus test effectively tells us if there is a statistically significant difference somewhere among our groups. However, suppose you were looking at different teaching methods’ effects on student performance. In that case, the omnibus test might reveal differences across all methods tested but won’t specify where those differences lie—which pairs or combinations of teaching methods vary significantly from one another.
The gist is this: though ANOVA can flag if at least two groups differ, it remains silent on the details. That’s like knowing you’ve got a winning lottery ticket without knowing its value—surely you’d want to dig deeper for specifics?
Understanding why post hoc tests are necessary
Digging into specifics is exactly where post hoc testing ANOVA steps up to bat. Once ANOVA waves a green flag signaling overall significance, we’re left with tantalizing questions: Which groups precisely account for these differences? Is every group distinct from each other, or are only specific ones driving the change?
Endeavoring to answer these queries without further assessment runs the risk of drawing inaccurate conclusions based on general trends rather than specific distinctions. Post hoc tests come equipped with a fine-comb approach that disaggregates data and provides granular insights into individual group comparisons after your initial ANOVA has pointed out broad variations among groups.
These follow-up evaluations pinpoint precisely which contrasts are significant, making them indispensable when crafting a nuanced understanding of your results.
The concept of experiment-wise error rate
A crucial underpinning principle in deciding when post hoc testing is imperative lies within what statisticians call “experiment-wise error rate.” This refers to the likelihood of committing at least one Type I error throughout all hypotheses tests performed within an experiment—not just per comparison but cumulatively over all possible post hoc pairwise comparison tests.
Imagine you’re tasting various batches of cookies trying to determine if any flavor stands out as more delicious. Each taste test increases the probability of incorrectly declaring one batch supreme due merely to chance—the more comparisons you make, the higher your risk of misjudgment because some findings could be false alarms.
Post hoc testing brings sophistication to our statistical toolkit by accounting for this cumulative error and controlling it using adjusted p-values—a procedure designed not only for added accuracy but also for confidence in our conclusions’ validity and reliability.
Different Post-Hoc Testing Methods
After performing an ANOVA, which tells you if there’s a statistically significant effect among group means, it’s quite common to wonder where the differences actually lie. That’s where post hoc testing comes in—think of it as peering closer into your data’s narrative to understand each character’s role. Let’s delve into this further with some methods that illuminate these nuanced stories.
Tukey’s Method
Explanation of Tukey’s method and its application in ANOVA
Tukey’s Honest Significant Difference (HSD) method is one of the most widely used post hoc tests following an ANOVA. When you’ve discerned that not all group means are equal, but you need to know which specific means differ, Tukey’s method steps up. It compares all possible pairs of means while controlling for the Type I error rate across those comparisons. This characteristic makes it particularly useful when you’re working with multiple groups and require multiple comparison tests a robust analysis.
Calculation and interpretation of adjusted p-values
Tukey’s method involves computing a set of “adjusted” p-values for each pair-wise comparison between group means. The calculation draws upon the studentized range distribution factoring in both within-and-between-group variances—all rather heady but central for interpreting the nuances within your data. What matters is that you adjust these p-values to account for the increased potential for Type I errors owing to multiple comparisons. If a particular adjusted p-value falls below the threshold of significance (usually 0.05), then voilà—you can declare a meaningful difference between those two group means.
Using simultaneous confidence intervals with Tukey’s method
Another powerful aspect of Tukey’s test includes its ability to create simultaneous confidence intervals for all mean differences. This visual representation of mean difference helps researchers not only see which groups differ but also understand the magnitude and direction of those differences—an invaluable insight when plotting future research or practical applications.
Holm’s Method
Introduction to Holm’s method and its advantages over other methods
Shifting gears, Holm’s method, known too as Holm’s sequential Bonferroni procedure, provides an alternative way of post hoc testing where conservation against Type I errors takes center stage—it adjusts p-values like a careful curator preserving valuable artifacts from undue exposure. Its most startling advantage lies in procedural flexibility; unlike some methods fixing upon single-step adjustments, Holm’s step-down approach offers more power while still playing defense against statistical flukes arising from many comparisons.
Calculation and interpretation of adjusted p-values with Holm’s method
The nitty-gritty involves ranking our initial unadjusted p-values from smallest to largest and subjecting them to sequential scrutiny against modified alpha levels based on their rank-order position—a kind of ‘stepping down’ process until we hit a value stubbornly larger than our computed threshold; cues are taken off at that point forward.
Dunnett’s Method
Explanation of Dunnett’s method and when it is appropriate to use it
Here we have Dunnett’s test, distinguished by its targeted approach: comparing multiple treatment groups specifically against a single control group—a common scenario in clinical trials or agronomic studies where you might want to weigh new treatments against a standard or placebo benchmark.
Comparing treatment groups to a control group using Dunnett’s method
Unlike other approaches casting wider nets across all possible comparisons, Dunnett’s discerning eyes only how each candidate stands up next to our chosen reference point. As such, it carefully calculates how much more leverage—or not—we’re getting out of your interventions versus doing nothing at all or sticking with what has been tried and true till now.
These various post hoc testing tools in ANOVA allow us statisticians and data analysts alike to divine detail from datasets brimming with potential insights just waiting beneath their numerical surfaces—each one tailored slightly differently toward revealing hidden stories woven into the fabric comprising our empirical inquiries.
Factors to Consider in Choosing a Post-hoc Test
When you venture into the realm of ANOVA, after identifying a significant difference across groups using an omnibus ANOVA test, the next step is often to employ post hoc testing to pinpoint exactly where those differences lie. Now, let me guide you through one of the critical factors that should influence which post hoc test you select: family-wise error rate control.
Famil-wise Error Rate Control and its Significance in Choosing a Test Method
The term ‘family-wise error rate’ (FWER) refers to the probability of making at least one Type I error among all possible comparisons when conducting multiple pairwise tests. A Type I error occurs when you incorrectly conclude differences exist between groups when, in truth, they do not. If not properly controlled, as we increasingly conduct more multiple pairwise comparisons within our ANOVA framework, the likelihood of inadvertently declaring a false significance balloons — potentially leading your study astray.
Even if that sounds daunting, fear not; it’s precisely why FWER control methods are crucial elements in selecting a post hoc test. Essentially these methods adjust your significance thresholds or p-values so that the collective risk across all tests does not exceed your original level of acceptance for errors (commonly 0.05). By doing this, we can explore specific group differences confidently without escalating our chances of false discoveries.
Controlling for FWER maintains the integrity of your findings and upholds the scientific rigor necessary for peer evaluation and reproducibility.
Now imagine you are faced with various post hoc testing options — understanding FWER helps you answer key questions:
- In my study design, how many comparisons will be made?
- How conservative do I need to be in controlling Type I errors considering my field or research question?
For example, Tukey’s HSD (Honestly Significant Difference) is best-suited when we’re making all possible, pairwise comparisons and comparisons and seeking to keep our family-wise error rate equal to our alpha level (often 0.05). Holm’s method steps up by adjusting p-values sequentially and striking a balance – it’s less conservative than Bonferroni but still offers reasonable protection against Type I errors. And if there’s a single control or reference group involved in your design? Dunnett’s method may come into play since it specifically addresses comparisons against that central figure.
In conclusion:
Effectively mitigating risks associated with increased hypothesis testing requires smart choices concerning statistical analysis methods. When diving headfirst into post hoc testing following an ANOVA result indicating significant variance across groups—remember always: Family-wise error rate control isn’t just statistical jargon; it’s your safeguard ensuring the reliability and validity of conclusions drawn from complex data patterns.
Case Studies and Examples
Understanding concepts in statistics is greatly enhanced by examining real-world applications. Let’s delve into how post hoc testing ANOVA breathes life into research studies, lending scientific inquiries a rigorous method for exploring their findings.
Discussion of real-world research studies where post hoc testing was used
Examined through the lens of practical application, post hoc analyses and tests become more than abstract mathematical procedures; they are tools that unfold narratives within data. For instance, a study focusing on the effectiveness of different teaching methodologies might employ an ANOVA to determine if there are significant differences in student outcomes based on the instructional approach. If the omnibus test yields a significant result, it paves the way for post hoc analysis—essential in pinpointing exactly which methods differ from each other.
Let me share another example that highlights this methodology: imagine researchers conducted a post hoc analysis of an experiment assessing a new medication’s impact on blood pressure levels. An initial ANOVA indicates that blood pressure readings vary significantly among different dosage groups over time. Post hoc testing steps in as a crucial next step, helping scientists compare every possible pair of dosages to understand specifically which ones are effective or potentially harmful.
These examples showcase how post hoc testing after ANOVA not only guides researchers through their discovery journey but also ensures robustness and precision in their conclusions.
Hands-on examples illustrating the application of different post hoc tests
Delving deeper into multiple comparison tests for specific applications can provide insight into how varied these tests can be:
- Tukey’s Method: Consider agricultural scientists comparing crop yields across multiple fertilizer types. Following a significant ANOVA finding different yields between treatments, Tukey’s method could reveal precisely which fertilizers yield statistically distinct crops compared to others—all while controlling for type I error across all comparisons.
- Holm’s Method: In psychological research aiming at understanding therapy outcomes, Holm’s sequential procedure would adjust p-values when multiple treatment forms are assessed against control groups. This ensures subsequent findings remain reliable even after discovering certain therapies outperform no treatment at all.
- Dunnett’s Method: Often used in clinical trials with a placebo group, Dunnett’s method contrasts each treatment directly against the placebo. A study evaluating several new pain relief drugs compared to placebo might utilize Dunnett’s to discern if any new drug has a superior effect without inflating the risk of false positives due to multiple comparisons.
These snippets from diverse fields underline how tailored post hoc testing in ANOVA gives substance to the lower statistical power of significance—transforming numbers into meaningful insights that can help shape industries and improve lives.
Statistical Power in Post-Hoc Testing
Explanation of statistical power and its importance in post hoc testing decision-making
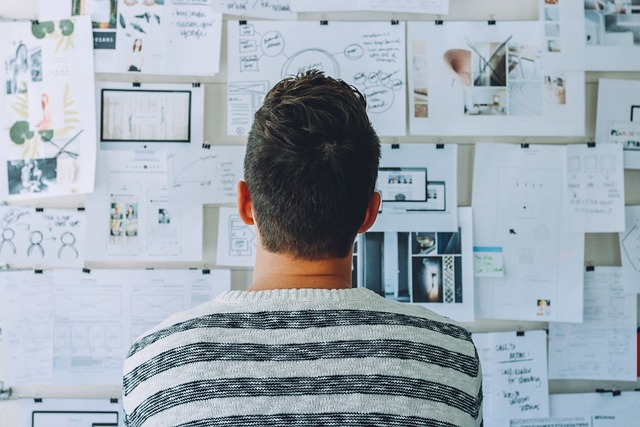
When discussing the intricacies of post hoc testing ANOVA results, it’s imperative to understand a concept at the heart of hypothesis testing—statistical power. In simpler terms, statistical power is the likelihood that a study will detect an effect when there truly is one. This translates to finding genuine differences between groups if they indeed exist.
High statistical power decreases the probability of committing a Type II error, which occurs when we fail to detect a difference that is actually present. It safeguards our results against false negatives, bolstering the reliability of conclusions drawn from our analysis. This factor becomes especially critical during post hoc tests after an ANOVA has suggested significant disparities across groups.
In practical settings, achieving high statistical power often means ensuring your study has an adequate sample size. While too small a sample might not reflect true group differences accurately, exceptionally large samples could reveal statistically significant but practically irrelevant differences. Henceforth, balancing these considerations is crucial for cogent decision-making in any research setting involving post hoc testing ANOVA.
Managing power trade-offs by reducing the number of comparisons
To address potential pitfalls inherent in multiple comparisons post-ANOVA, researchers ought to judiciously manage the compromise between maintaining sufficient statistical power and controlling for an inflated risk of type I errors (false positives). Here are effective strategies:
- Prioritization: Determine which comparisons are most vital to your hypotheses and prioritize those for further scrutiny.
- Consolidation: Instead of examining all possible pairwise comparisons among treatment levels, focus only on comparing each treatment group with control or combine treatment groups into meaningful categories.
By thoughtfully selecting fewer comparisons, researchers not only boost the chances that their study retains robust statistical strength but also reduce the experiment-wise error rate without overwhelming correction procedures eating into their discovery potential.
Handling this delicate equilibrium astutely ensures that substantively important findings stand out while still affirming methodological rigor—an essential balance point for all studies employing post hoc testing following an ANOVA framework.
Summary and Conclusion
Recap of key points covered in the content outline
Throughout this article, we’ve traversed the landscape of Analysis of Variance (ANOVA) and its critical companion – post hoc testing ANOVA. To start, we established a foundational understanding of ANOVA, where it’s used to discern whether there are any statistically significant differences between the means of three or more independent groups.
We dug into the intricacies of post hoc testing, which is essential when an initial ANOVA yields significant results. We identified that while an ANOVA can tell us that at least two groups differ, it doesn’t specify which groups or how many differ from each other. That’s where post hoc tests come in.
The journey took us through various twists and turns as we discussed:
- The critical nature of ANOVA’s omnibus test which uses the F-statistic to determine overall variance.
- The significance of interpreting these results accurately for sound statistical analysis.
When limitations such as experiment-wise error rates revealed themselves, we understood why post hoc testing is not just helpful but necessary. It offers refined insights by controlling these error rates and allowing for multiple comparisons without inflating the likelihood of type I errors.
In our expedition through different methods like Tukey’s, Holm’s, and Dunnett’s, you probably noticed they serve unique purposes—be it comparing multiple comparisons of all possible pairs of means or focusing on a single control group comparison.
Choosing a post hoc test invokes careful consideration. Error rate control doesn’t happen in isolation; howell post hoc tests, one must weigh factors related to family-wise error rates.
Lacing real-world examples into our discussion helped ground these conceptual considerations solidly within practical application scenarios.
Finally, yet importantly, we touched upon statistical power. While reducing the number of comparisons is sometimes seen as reducing power trade-offs’, strategic decision-making here ensures robustness in findings even when engaging with multiple post hoc tests here.
Concluding thoughts on the importance and significance of post hoc testing in ANOVA
To conclude this insightful excursion into post hoc testing ANOVA, let’s remind ourselves why diving deep into this particular territory of statistical analysis matters so greatly. In research contexts spanning from healthcare breakthroughs to groundbreaking technology developments, ensuring that our findings are not just statistically relevant but also practically significant can make all the difference.
The judicious use of post hoc tests following an ANOVA allows us to step beyond mere detection of differences and venture into exploring what those differences are—and their size—with precision and confidence impactful enough to influence subsequent research paths decisively or policy decisions effectively.
As eager scholars and dedicated professionals navigating an increasingly data-driven world, approaches like these don’t just refine our understanding—they expand possibilities. Post hoc tests continue holding a torch high illuminating nuanced details amidst sometimes overwhelming datasets—a beacon guiding towards conclusive insights magnifying our ability to make informed decisions based on robust analytical processes that stand up against scrutiny ardently both within scientific circles and out on fields pioneering innovations earnestly pursued for societal benefits multidimensional in scope true to what inspires each new search ‘..for patterns unforeseen.’
Through it all, my hope remains steadfast: may your own analyses yield fruitful understanding interspersed with clarity deserving of accolades ultimately improving lives touched by evidence-based practices standing timelessly testamentary upon rigorous statistical grounds defining distinction tirelessly enduring…in pursuit of truth ever elusive yet eternally enticing.
Experience the Power of Visual Mastery: Simplifying Complexity with Mind the Graph!
Unlock the potential of flawless visual communication as we redefine the way you comprehend intricate concepts. In an era dominated by visuals, understanding complex ideas, even something as enigmatic as quantum physics, becomes a breeze through the sheer effectiveness of graphics.
Embark on your visual journey with Mind the Graph, your ultimate companion in transforming complex messages into captivating visuals. With over a thousand meticulously crafted illustrations in our gallery, the possibilities are limitless. Our cutting-edge smart poster maker empowers you to effortlessly create posters that stand out.
Why settle for the ordinary when you can have a tailored visual masterpiece? Harness the expertise of our talented team to customize illustrations according to your unique needs. Mind the Graph is not just a tool; it’s your gateway to a world where visuals speak louder than words.
Ready to amplify your communication game? Sign up for free and start creating now. Your message, our visuals – a flawless combination!
Subscribe to our newsletter
Exclusive high quality content about effective visual
communication in science.