The role of Artificial Intelligence (AI) in academic research has garnered significant attention in recent years. This transformative technology, powered by machine learning algorithms and data analytics, is revolutionizing the research landscape. By enabling researchers to process vast amounts of data, extract meaningful insights, and automate repetitive tasks, AI has the potential to accelerate the pace of scientific discovery and enhance the quality of research outcomes.
As AI continues to evolve, it is essential for researchers to adapt and embrace this powerful tool while also being mindful of its limitations and ethical implications. By striking a balance between AI-driven automation and human ingenuity, researchers can unlock new possibilities, advance scientific knowledge, and contribute to the transformative potential of AI in the realm of academic research.
How does AI change academia?
AI has brought about significant changes to academia, revolutionizing the way research is conducted, knowledge is generated, and education is delivered. The integration of AI technologies in academia has the potential to streamline processes, enhance research outcomes, and foster innovation.
One of the primary ways AI is changing academia is through data analysis. Researchers can leverage AI algorithms to analyze vast amounts of data quickly and efficiently. This enables them to identify patterns, correlations, and trends that may not be easily discernible through traditional methods.
Furthermore, AI is transforming the research process itself. It can assist researchers in literature review and knowledge synthesis by automatically scanning and extracting relevant information from a wide range of scientific papers. This not only saves time but also helps researchers stay up-to-date with the latest advancements in their field.
Another area where AI is making a significant impact in academia is in education. AI-powered technologies are being used to develop intelligent tutoring systems, adaptive learning platforms, and personalized educational experiences. These technologies can analyze students’ learning patterns and provide tailored feedback, support, and resources.
Moreover, AI has the potential to augment human capabilities in academia. It can automate repetitive tasks, freeing up researchers’ time to focus on higher-level cognitive activities. This includes automating data collection, analysis, and even manuscript writing. By streamlining these processes, researchers can devote more time to critical thinking, hypothesis generation, and exploring new research avenues.
To gain a deeper understanding of the role of AI in science and explore its transformative potential, we highly encourage readers to delve into the article “Artificial Intelligence in Science” published on Mind the Graph’s blog.
Application of AI in academic research
Artificial intelligence has found numerous applications in academic research across various disciplines. Here are some examples of how AI is being used in academic research:
- Data analysis and pattern recognition: AI algorithms can analyze large datasets and identify patterns, correlations, and trends that may not be easily recognizable by humans alone. This is particularly useful in fields such as genomics, climate science, and social sciences.
- Natural language processing (NLP): NLP techniques enable computers to understand and generate human language. Researchers use NLP to analyze large volumes of textual data, extract information, summarize documents, and detect sentiment. It has applications in fields like literature, linguistics, and social sciences.
- Computer vision: AI-based computer vision systems can process and interpret visual data, such as images and videos. Researchers use computer vision to analyze medical images, satellite imagery, and surveillance footage, among others. It has applications in fields like biology, astronomy, and environmental sciences.
- Drug discovery and development: AI is being used to accelerate the process of drug discovery by predicting the properties and interactions of potential drug compounds. Machine learning models can analyze vast amounts of chemical and biological data to identify potential drug targets and design novel molecules.
- Robotics and automation: AI-powered robots and automated systems are increasingly being used in academic research to perform tasks such as lab experiments, data collection, and sample processing. These robots can work 24/7, reducing human error and increasing efficiency in research workflows.
- Recommendation systems: AI algorithms can provide personalized recommendations based on user preferences and behaviors. In academia, these systems can suggest relevant research papers, conferences, or collaborations based on a researcher’s interests and previous work.
- Simulation and modeling: AI techniques, such as machine learning and neural networks, can be used to create complex models and simulations. Researchers can use these models to study and predict phenomena in fields like physics, economics, and social sciences.
- Knowledge discovery and synthesis: AI can assist researchers in discovering and synthesizing information from vast amounts of existing research papers, patents, and other academic sources. This can help identify research gaps, find relevant literature, and generate new insights.
Challenges and Ethical Considerations in AI-Driven Academic Research
While AI-driven academic research offers significant benefits, there are also several challenges and ethical considerations that researchers need to address. Here are some of the key challenges and ethical considerations associated with AI in academic research:
- Data bias and fairness: AI systems are trained on data, and if the training data is biased or reflects societal prejudices, the AI models can perpetuate those biases. Researchers need to carefully curate and preprocess data to ensure fairness and mitigate bias in AI models.
- Privacy and data protection: AI research often involves handling large amounts of data, including personal and sensitive information. Researchers must ensure that data collection, storage, and analysis adhere to relevant privacy regulations and obtain informed consent from participants.
- Transparency and interpretability: Some AI algorithms, such as deep learning models, can be considered black boxes, making it difficult to understand and interpret their decision-making processes. In academic research, it is important to strive for transparency and develop methods to explain the reasoning behind AI-driven results.
- Reproducibility and robustness: Researchers should aim for reproducibility by providing clear documentation of their AI models, algorithms, and datasets. It is crucial to ensure that AI models are robust and can generalize well to unseen data, avoiding overfitting or biased results.
- Intellectual property and ownership: AI research often involves collaboration and the use of pre-existing datasets and models. Clear guidelines need to be established regarding intellectual property rights, data ownership, and the sharing of AI models and code among researchers.
- Accountability and liability: As AI becomes more autonomous, questions of accountability and liability arise. Researchers must consider the ethical implications of their AI systems and be aware of the potential risks and consequences associated with their deployment.
- Social impact and job displacement: AI technologies have the potential to disrupt industries and automate certain job roles. Researchers should be mindful of the social impact of their AI-driven research and work towards ensuring a just transition, job creation, and minimizing negative consequences.
- Dual-use and misuse: AI technologies developed for academic research can have both positive and negative applications. Researchers should be mindful of potential dual-use scenarios and consider the ethical implications of their work to prevent misuse or unintended harm.
The Future of AI in Academic Research
The future of AI in academic research holds immense potential for transformative advancements. Here are some trends, opportunities, and potential impacts to consider:
- Interdisciplinary collaboration: AI brings together researchers from different disciplines, fostering collaboration and enabling breakthrough insights.
- Data-driven discoveries: AI algorithms extract valuable insights from large datasets, revolutionizing research across disciplines.
- Personalized and adaptive learning: AI technologies provide tailored educational experiences, assessing student performance and offering targeted feedback.
- Enhanced scientific discovery: AI assists researchers in hypothesis generation, experiment design, and data analysis, accelerating the research process.
- Ethical considerations and responsible AI: Researchers address bias, transparency, privacy, and accountability to ensure ethical and responsible AI use.
- AI-enabled automation: AI streamlines research workflows, automating tasks like data collection and analysis, improving efficiency.
- AI for global challenges: AI contributes to solving climate change, healthcare, and poverty by analyzing data and optimizing resource allocation.
- Augmented creativity: AI serves as a creative partner, generating ideas, synthesizing information, and pushing boundaries in fields like art and design.
- Enhanced peer review and scientific communication: AI automates aspects of peer review, aids in language translation, and recommends relevant research papers.
- Democratization of research: AI platforms provide access to computational power, datasets, and collaboration opportunities globally, democratizing research.
AI Tools for Academic Research
- Pictory: Pictory is an AI-powered video generator that simplifies the process of creating and editing high-quality videos.
- Jasper: Jasper stands out as the top AI writing assistant, setting the standard in the market with its exceptional features and remarkable quality.
- Murf: Murf, the text-to-speech generator, is widely recognized as one of the most popular and remarkable AI voice generators available in the market.
- HitPaw Photo Enhancer: AI-based tool for enhancing image quality and details.
- ChatGPT: AI model for natural language processing and generating human-like text responses.
- Lovo.ai: Lovo.ai has garnered accolades as an award-winning voice generator and text-to-speech solution.
- Reply.io: Reply offers a comprehensive sales engagement platform that enables the scalable creation of new opportunities while ensuring a personalized touch in every interaction.
Exclusive scientific content created by scientists
Mind the Graph is a platform built by scientists for scientists, designed to assist in creating visually appealing scientific content. It offers customizable illustrations, templates, and graphs, empowering researchers to communicate their findings effectively. With collaboration features, a presentation mode, and seamless export and integration options, scientists can create engaging content for academic publications and presentations. The platform also provides learning resources, supporting scientists in enhancing their visual communication skills.
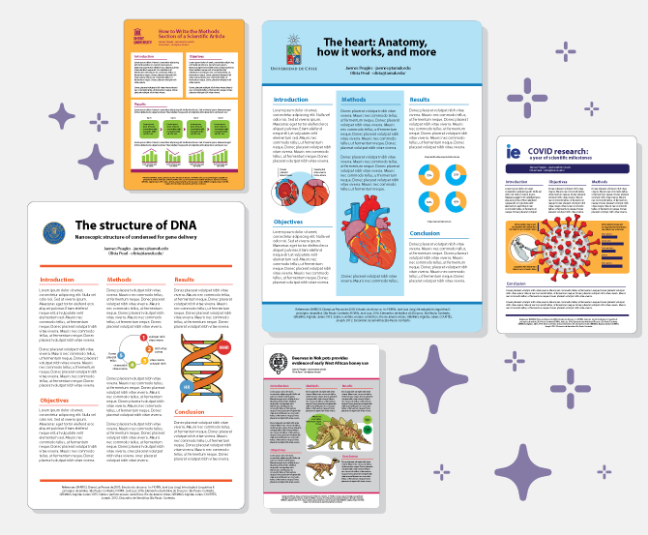
Subscribe to our newsletter
Exclusive high quality content about effective visual
communication in science.