When it comes to data analysis, accuracy is everything. Misclassification bias is a subtle yet critical issue in data analysis that can compromise research accuracy and lead to flawed conclusions. This article explores what misclassification bias is, its real-world impact, and practical strategies to mitigate its effects. Inaccurate categorization of data can lead to flawed conclusions and compromised insights. We will explore what misclassification bias is, how it impacts your analysis, and how to minimize these errors to ensure reliable results in the following.
Understanding the Role of Misclassification Bias in Research
Misclassification bias occurs when data points such as individuals, exposures, or outcomes are inaccurately categorized, leading to misleading conclusions in research. By understanding the nuances of misclassification bias, researchers can take steps to improve data reliability and the overall validity of their studies. Because the data being analyzed does not represent the true values, this error can lead to inaccurate or misleading results. A misclassification bias occurs when participants or variables are categorized (e.g., exposed vs. unexposed, or diseased vs. healthy). It leads to incorrect conclusions when subjects are misclassified, as it distorts the relationships between variables.
It is possible that the results of a medical study that examines the effects of a new drug will be skewed if some patients who are actually taking the drug are classified as “not taking the drug,” or vice versa.
Types of Misclassification Bias and Their Effects
Misclassification bias can manifest as either differential or non-differential errors, each impacting research outcomes differently.
1. Differential Misclassification
When misclassification rates differ between study groups (for example, exposed vs. unexposed, or cases vs. controls), this occurs. The errors in classification vary based on which group a participant belongs to, and they are not random.
During a survey on smoking habits and lung cancer, if the smoking status is misreported more frequently by people suffering from lung cancer due to social stigmas or memory problems, this would be considered differential misclassification. Both the disease status (lung cancer) and the exposure (smoking) contribute to the error.
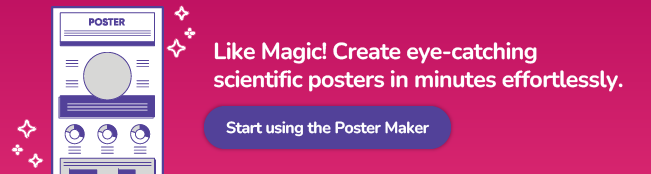
It is often the case that differential misclassification results in a bias toward the null hypothesis or away from it. Because of this, the results may exaggerate or underestimate the true association between the exposure and the outcome.
2. Non-Differential Misclassification
A non-differential misclassification occurs when the misclassification error is the same for all groups. As a result, the errors are random, and the misclassification does not depend on exposure or outcome.
In a large-scale epidemiological study, if both cases (people with the disease) and controls (healthy individuals) report their diets incorrectly, this is called non-differential misclassification. Regardless of whether participants have the disease or not, the error is equally distributed between the groups.
The null hypothesis is typically favored by non-differential misclassification. Therefore, any real effect or difference is harder to detect since the association between variables is diluted. It is possible for the study to conclude incorrectly that there is no significant relationship between the variables when there is actually one.
Real-World Implications of Misclassification Bias
- Medical Studies: In research on the effects of a new treatment, if patients who don’t receive the treatment are mistakenly recorded as having received it, the efficacy of the treatment could be misrepresented. Diagnostic errors can also skew results, where a person is wrongly diagnosed with a disease.
- Epidemiological Surveys: In surveys assessing exposure to hazardous substances, participants might not accurately recall or report their exposure levels. When asbestos-exposed workers underreport their exposure, it can lead to misclassification, changing the perception of asbestos-related disease risks.
- Public Health Research: When studying the relationship between alcohol intake and liver disease, participants who drink heavily would be misclassified as moderate drinkers if they underreported their intake. This misclassification could weaken the observed association between heavy drinking and liver disease.
In order to minimize the effects of misclassification bias, researchers must understand its type and nature. Studies will be more accurate if they recognize the potential for these errors, regardless of whether they are differential or non-differential.
Impact of Misclassification Bias on Data Accuracy
Misclassification bias distorts data accuracy by introducing errors in variable classification, jeopardizing the validity and reliability of research results. Data that does not accurately reflect the true state of what is being measured can lead to inaccurate conclusions. When variables are misclassified, whether by putting them in the wrong category or incorrectly identifying cases, it can lead to flawed datasets that jeopardize the overall validity and reliability of the research.
Impact on Validity and Reliability of Study Results
A study’s validity is compromised by misclassification bias since it skews the relationship between variables. For example, in epidemiological studies where researchers are assessing the association between an exposure and a disease, if individuals are incorrectly classified as having been exposed when they have not, or vice versa, the study will fail to reflect the true relationship. This leads to invalid inferences and weakens the conclusions of the research.
Misclassification bias can also affect reliability, or the consistency of results when repeated under the same conditions. Performing the same study with the same approach may yield very different results if there is a high level of misclassification. Scientific research is based on confidence and reproducibility, which are essential pillars.
Misclassification Can Lead to Skewed Conclusions
- Medical Research: In a clinical trial examining the effectiveness of a new drug, if patients are misclassified in terms of their health status (e.g., a sick patient is classified as healthy or vice versa), the results could falsely suggest that the drug is either more or less effective than it truly is. An incorrect recommendation about the drug’s use or efficacy could lead to harmful health outcomes or the rejection of potentially life-saving therapies.
- Survey Studies: In social science research, particularly in surveys, if participants are misclassified due to errors in self-reporting (e.g., misreporting income, age, or education level), the results may produce skewed conclusions about societal trends. It is possible that flawed data can influence policy decisions if low-income individuals are incorrectly classified as middle-income in a study.
- Epidemiological Studies: In public health, misclassification of diseases or exposure status can dramatically alter study results. Incorrectly categorizing individuals as having a disease will overestimate the prevalence of that disease. A similar problem can occur if the exposure to a risk factor is not properly identified, leading to an underestimation of the risk associated with the factor.
Causes of Misclassification Bias
Data or subjects are misclassified when they are categorized into the wrong groups or labels. Among the causes of these inaccuracies are human error, misunderstandings of categories, and the use of faulty measurement tools. These key causes are examined in more detail below:
1. Human Error (Inaccurate Data Entry or Coding)
Misclassification bias is frequently caused by human error, particularly in studies that rely on manual data entry. Typos and misclicks can result in data being entered into the wrong category. A researcher might erroneously classify a patient’s disease status in a medical study, for instance.
Researchers or data entry personnel may use inconsistent coding systems to categorize data (e.g., using codes like “1” for males and “2” for females). It is possible to introduce bias if coding is done inconsistently or if different personnel use different codes without clear guidelines.
A person’s likelihood of making mistakes increases when they are fatigued or pressed for time. Misclassifications can be exacerbated by repetitive tasks like data entry, which can lead to lapses in concentration.
2. Misunderstanding of Categories or Definitions
Defining categories or variables in an ambiguous way can lead to misclassification. Researchers or participants can interpret a variable differently, leading to inconsistent classification. The definition of “light exercise” might differ considerably between people in a study on exercise habits, for example.
Researchers and participants may find it difficult to differentiate between categories when they are too similar or overlapped. Data may be classified incorrectly as a result of this. The distinction between the early and mid stages of a disease might not always be clear-cut when studying various stages.
3. Faulty Measurement Tools or Techniques
Instruments that are not accurate or reliable can contribute to misclassification. Data classification errors can occur when faulty or improperly calibrated equipment gives incorrect readings during physical measurements, such as blood pressure or weight.
There are times when tools work fine, but measurement techniques are flawed. As an example, if a healthcare worker does not follow the correct procedure for collecting blood samples, inaccurate results may result and the health status of the patient could be misclassified.
Machine learning algorithms and automated data categorization software, when not properly trained or prone to errors, can also introduce bias. The study results might be systematically biased if the software does not account for edge cases correctly.
Effective Strategies to Address Misclassification Bias
Minimizing misclassification bias is essential for drawing accurate and reliable conclusions from data, ensuring the integrity of research findings. The following strategies can be used to reduce this type of bias:
Clear Definitions and Protocols
It is common for variables to be misclassified when they are poorly defined or ambiguous. All data points must be defined precisely and unambiguously. Here’s how:
- Make sure that categories and variables are mutually exclusive and exhaustive, leaving no room for interpretation or overlap.
- Create detailed guidelines that explain how to collect, measure, and record data. This consistency reduces variability in data handling.
- Check for misunderstandings or gray areas by testing your definitions with real data through pilot studies. Modify definitions as necessary based on this feedback.
Improving Measurement Tools
A major contributor to misclassification bias is the use of faulty or imprecise measurement tools. Data collection is more accurate when tools and methods are reliable:
- Make use of tools and tests that have been scientifically validated and are widely accepted in your field. By doing so, they ensure both the accuracy and comparability of the data they provide.
- Check and calibrate instruments periodically to ensure that they provide consistent results.
- You can reduce classification errors by using scales with greater precision if your measurements are continuous (e.g., weight or temperature).
Training
Human error can significantly contribute to misclassification bias, especially when those collecting the data are not fully aware of the requirements or nuances of the study. Proper training can mitigate this risk:
- Provide detailed training programs for all data collectors, which explain the purpose of the study, the importance of correct classification, and how variables should be measured and recorded.
- Provide ongoing education to ensure that long-term study teams remain familiar with protocols.
- Ensure that all data collectors understand the processes and can apply them consistently after training.
Cross-validation
To ensure accuracy and consistency, cross-validation compares data from multiple sources. Errors can be detected and minimized using this method:
- Data should be collected from as many independent sources as possible. Discrepancies can be identified by verifying the accuracy of the data.
- Identify any potential inconsistencies or errors in collected data by cross-checking it with existing records, databases, or other surveys.
- The replication of a study or part of a study can sometimes help to validate the findings and reduce misclassification.
Rechecking Data
It is essential to continuously monitor and recheck data after collection in order to identify and correct misclassification errors:
- Implement real-time systems for detecting outliers, inconsistencies, and suspicious patterns. By comparing entries against expected ranges or predefined rules, these systems can detect errors early on.
- When manual data entry is involved, a double-entry system can reduce errors. Discrepancies can be identified and corrected by comparing two independent entries of the same data.
- An annual audit should be performed to ensure that the data collection process is accurate and that protocols are followed.
These strategies can help researchers reduce the likelihood of misclassification bias, ensuring their analyses are more accurate and their findings are more reliable. Errors can be minimised by following clear guidelines, using precise tools, training staff, and performing thorough cross-validation.
Browse Through 75,000+ Scientifically Accurate Illustrations In 80+ Popular Fields
Understanding misclassification bias is essential, but effectively communicating its nuances can be challenging. Mind the Graph provides tools to create engaging and accurate visuals, helping researchers present complex concepts like misclassification bias with clarity. From infographics to data-driven illustrations, our platform empowers you to translate intricate data into impactful visuals. Start creating today and enhance your research presentations with professional-grade designs.
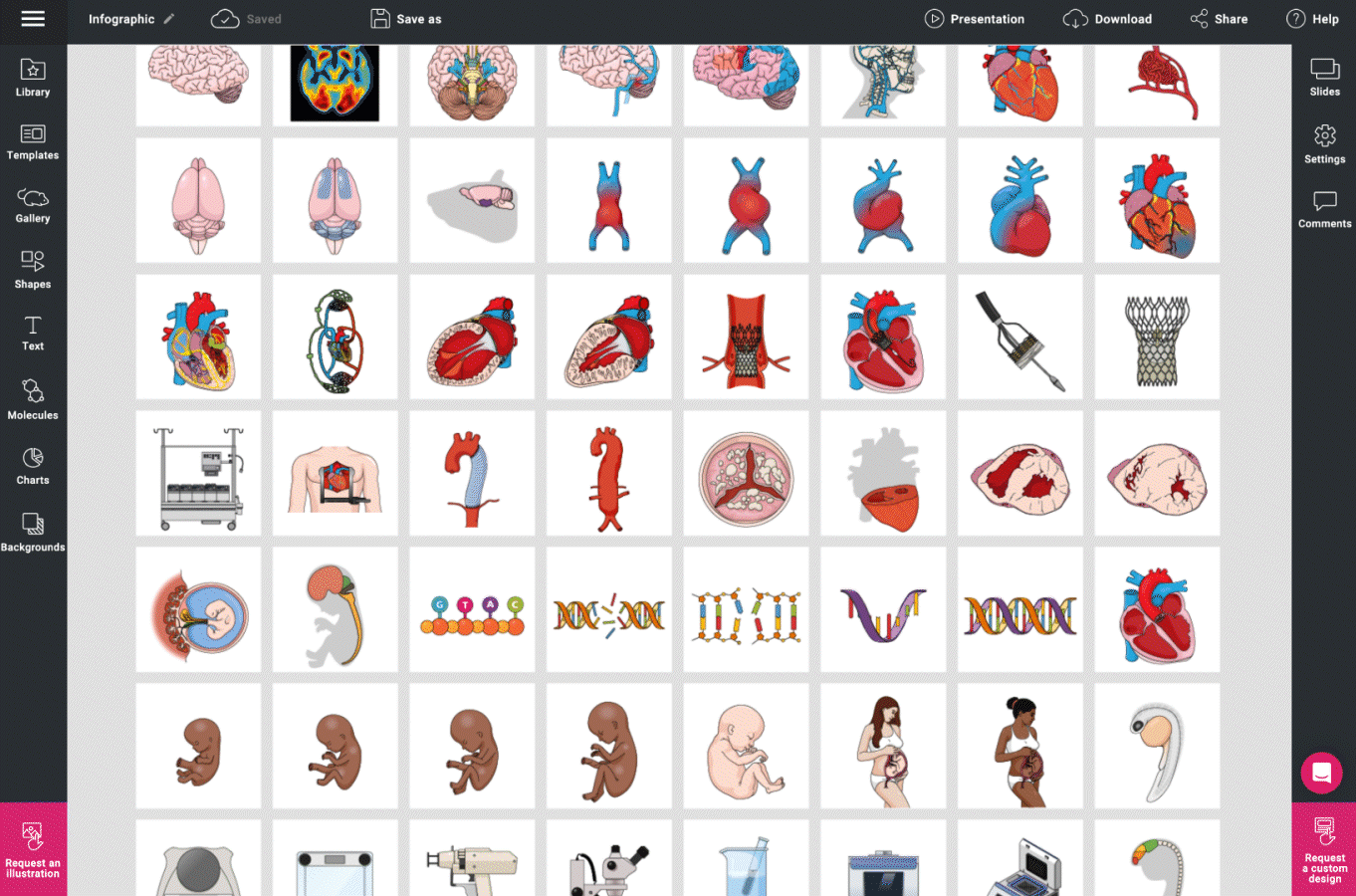
Subscribe to our newsletter
Exclusive high quality content about effective visual
communication in science.