The chi-square test is a powerful tool in statistics, especially for analyzing categorical data across various forms and disciplines. In some datasets, continuous numbers represent the data, while in others, categorical data represents the data grouped according to gender, preferences, or educational level. When analyzing categorical data, the chi-square test is a widely used statistical tool for exploring relationships and drawing meaningful insights. This article dives into how the chi-square test works, its applications, and why it’s essential for researchers and data analysts.
Throughout this blog, we will examine how the Chi-square test works, how it is performed, and how it can be interpreted. You can use the Chi-square test to better understand data analysis, whether you’re a student, researcher, or interested in data analysis in general.
Understanding the Importance of the Chi-square Test
The chi-square test is a fundamental statistical method used to examine relationships between categorical variables and test hypotheses in various fields. Understanding how to apply the chi-square test can help researchers identify significant patterns and associations in their data. Under the null hypothesis, it compares observed data to what we would expect if there were no relationship between the variables. In fields such as biology, marketing, and social sciences, this test is especially useful for testing hypotheses about population distributions.
At its core, the Chi-square test measures the discrepancy between observed and expected frequencies in categorical data. By using it, we can answer questions like: “Do the observed data patterns differ from what would be expected by chance?” or “Are two categorical variables independent of one another?”
Types of Chi-square Tests
The chi-square test comes in two primary forms—goodness of fit and independence tests—each tailored for specific statistical inquiries.
1. Chi-square Goodness of Fit Test
An individual categorical variable is tested to determine whether it follows a particular distribution. A model or historical data is often used to check if the observed data matches an expected distribution.
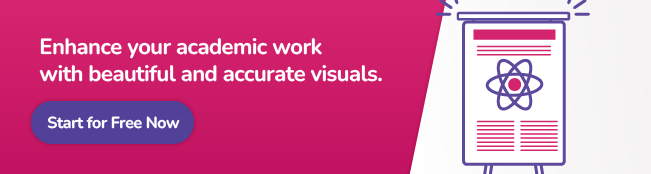
Think about rolling a die 60 times. Since the die is fair, you would expect each side to appear ten times, but the actual results vary slightly. In order to determine whether this deviation is significant or merely a result of chance, you can perform the goodness of fit test.
Steps Involved:
- Based on the theoretical distribution, determine the expected frequencies.
- Then compare them with the observed frequencies.
- Compute the Chi-square statistic to quantify the deviation.
Researchers often use this test in quality control, genetics, and other fields where they want to compare observed data with a theoretical distribution.
2. Chi-square Test of Independence
In this test, two categorical variables are evaluated for their independence. This test examines whether one variable’s distribution varies across levels of a second variable. Contingency tables, which display variables’ frequency distributions, are typically tested for independence using the Chi-square test.
Assume you conduct a survey asking participants about their gender and their preferred type of movie (action, drama, comedy). A Chi-square test of independence can be used to determine whether gender influences movie preferences or if they are independent.
Steps Involved:
- Create a contingency table for the two variables.
- Based on the assumption that the variables are independent, calculate the expected frequencies.
- Using the Chi-square statistic, compare the observed frequencies with the expected frequencies.
In market research, healthcare, and education, this test is widely used to study the relationship between demographic variables and outcomes, such as the relationship between education level and voting preferences.
Applications of the Chi-square Test in Real-World Scenarios
The chi-square test is particularly useful when working with categorical data, such as gender, preferences, or political affiliations, to test relationships and patterns. Tests of independence and goodness of fit are used to determine whether there is a significant association between two variables (test of independence).
Researchers can test hypotheses and determine patterns using the Chi-square test in categorical data. There are several reasons why it is widely adopted:
- Unlike parametric tests, it does not require assumptions about the distribution underlying the data.
- Various disciplines can use it, making it versatile.
- Based on observed patterns, it assists in making informed decisions.
Assumptions of the Chi-square Test
To ensure the validity of the Chi-square test results, certain assumptions must be met. These assumptions help maintain the accuracy and relevance of the test, especially when working with categorical data. Three key assumptions need to be addressed: random sampling, categorical variables, and expected frequency counts.
1. Random Sampling
Data must be collected through random sampling as the first and most fundamental assumption. As a result, the sample includes each individual or element equally. A random sample minimizes bias, so the results can be generalized to a larger population.
If the sample is not random, the results might be skewed, leading to incorrect conclusions. The results of a survey distributed exclusively to a specific group within a population may not reflect the views of the entire organization, thus violating the random sampling assumption.
2. Categorical Variables
Analyzing categorical variables – data that can be divided into distinct categories – is the purpose of the Chi-square test. There should be no numerical variables (although they can be coded numerically for convenience) and they should be grouped into clearly defined groups.
Examples of categorical variables include:
- Gender (male, female, non-binary)
- Marital status (single, married, divorced)
- Eye color (blue, brown, green)
A Chi-square test cannot be used directly with continuous data, such as height or weight, unless they are converted into categories. In order for the Chi-square test to be meaningful, the data must be categorical, such as “short,” “average,” or “tall.”
3. Expected Frequency Count
Another critical assumption of the Chi-square test is the expected frequency of the categories or cells in the contingency table. Assuming the null hypothesis is true (i.e. that the variables are not associated), the expected frequency is the theoretical frequency count that exists in each category.
The rule of thumb is that: The expected frequency for each cell should be at least 5. A low expected frequency can lead to unreliable results if the test statistic is distorted. Fisher’s Exact Test should be considered when expected frequencies fall below 5, especially in small sample sizes.
Step-by-Step Guide to Performing a Chi-square Test
- Setting up Hypotheses (Null and Alternative)
- Null Hypothesis (H0): There is no connection between the two things you are comparing. Any differences you see are just random.
- Alternative Hypothesis (H₁): This means there is a real connection between the two things. The differences are not random, but meaningful.
2. Creating the Contingency Table
Contingency tables show how often certain things occur together. The table, for instance, shows different groups (like men and women) and different choices (like which product they prefer). As you look at the table, you will see how many people fall into each of the groups and choices.
3. Calculating Expected Frequencies
If there were no real connection between the things you’re comparing, the expected frequencies would be what you would expect. A simple formula can be used to calculate them:
Expected Frequency = (Row Total × Column Total) /Grand Total
This just tells you what the numbers should look like if everything was random.
4. Computing the Chi-square Statistic
The chi-square test allows you to measure how much your observed data deviates from expected outcomes, helping determine if relationships exist. It looks complicated, but it compares the real numbers with those expected:
𝜒2=∑(Observed−Expected)2/ Expected
You do this for every box in your table and then add them all together to get one number, which is your Chi-square statistic.
5. Determining Degrees of Freedom
In order to interpret your results, you need to know the degrees of freedom. Based on your table’s size, you calculate them. Here is the formula:
Degrees of Freedom = ( Number of Rows −1)×(Number of Columns−1)
This is just a fancy way of accounting for the size of your data.
6. Using the Chi-square Distribution to Find the p-value
A p-value can be calculated using the Chi-square statistic and the degrees of freedom. When you look at the p-value, you can determine whether the differences you observed were likely due to chance or whether they were meaningful.
Interpreting the p-value:
- Usually, a small p-value indicates that the differences you found aren’t random, so you reject the null hypothesis. You can see a real connection between what you’re studying and what you’re doing.
- A p-value bigger than 0.05 indicates that the differences are likely random, so you should keep the null hypothesis. Therefore, there is no real connection between the two.
If two things happen by accident, or are related, you can use this simplified process to determine if they are connected!
Interpreting Results from the Chi-square Test
A Chi-square statistic tells us how much the actual data (what you observed) differs from what we would expect if there were no relationship between the categories. Essentially, it measures how far our observed results differ from what we predicted by chance.
- Big Chi-square value: The difference between your expectation and reality is large. It could indicate that something interesting is happening in your data.
- Small Chi-square value: This means the observed data is pretty close to what was expected, and there may not be anything unusual going on.
Although this is true, the Chi-square value alone does not provide you with all the information you need. Using a p-value, you can determine if a difference is significant or just a coincidence.
What the p-value Means
P-values help you determine whether the differences between your data are meaningful. In other words, it tells you what the probability is that the differences you observed are the result of random chance.
- Low p-value (typically 0.05 or less): This means the difference is unlikely to be due to chance. That is, there is probably a real difference, and something interesting is happening. As a result, you would reject the notion that there is no relationship (the “null hypothesis”).
- High p-value (greater than 0.05): This suggests that the difference could easily be due to chance. As a result, there’s no strong indication that anything unusual is occurring in your data. If there is no relationship between the categories, you would not reject the null hypothesis.
How to Draw Conclusions
Once you have both the Chi-square statistic and the p-value, you can draw conclusions:
Look at the p-value:
- You reject the idea that there is no relationship between two categories if the p-value is 0.05 or less. As an example, if you examine whether gender affects product preference and the p-value is low (0.05 or less), you can say: “It seems that gender affects people’s choices.”.
- If the p-value is more than 0.05, the data don’t show any significant difference, so you conclude that the categories are likely unrelated. Using a high p-value (greater than 0.05), you could say: “There isn’t any strong evidence that gender influences product preferences.
Remember real-world relevance
You should consider whether a statistically significant difference matters in real life, even if it shows a statistically significant difference. It is possible to consider even tiny differences important with a very large data set, but they may not have a significant impact in the real world. Rather than just looking at the numbers, always consider what the result means in practice.
It tells you whether the difference between what you expected and what you got is real or just a fluke, using a Chi-square statistic. You can determine if your data has a meaningful relationship when you combine them.
Visualizing Chi-square Test Results with Mind the Graph
The chi-square test helps uncover patterns in data, but presenting these insights effectively requires engaging visuals. Mind the Graph provides intuitive tools to create stunning visuals for your chi-square test results, making complex data easier to understand. Whether for academic reports, presentations, or publications, Mind the Graph helps you convey statistical insights with clarity and impact. Explore our platform today to transform your data into compelling visual stories.
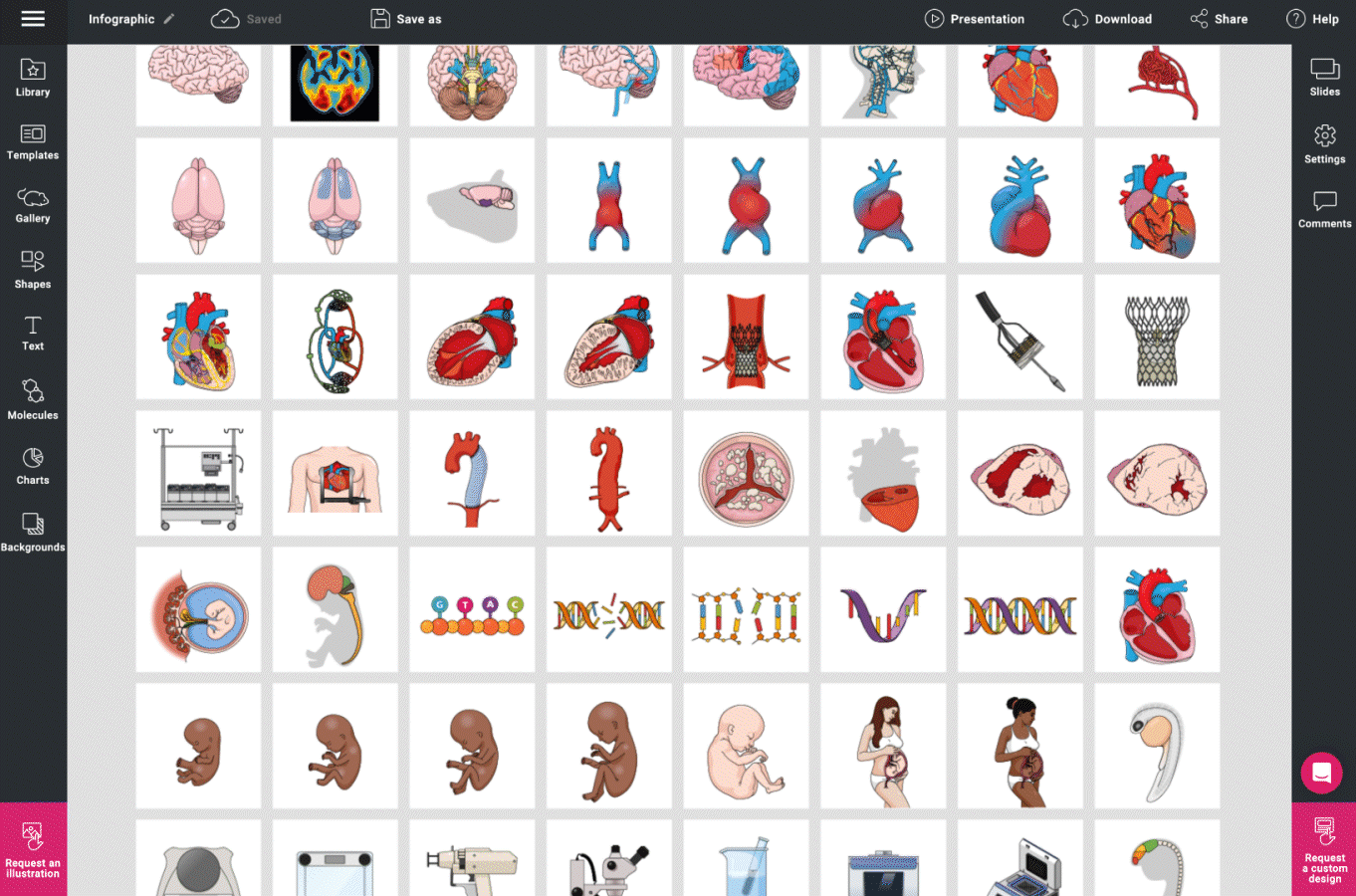
Subscribe to our newsletter
Exclusive high quality content about effective visual
communication in science.