Whenever you plan a layout for a building or create an emergency plan, it is quite important for you to know the geographic location of the place. Most people fail to notice the surroundings, rather they focus only on the building and its infrastructure. This is the reason why in recent days, there are more building collapses because the planner didn’t take the location in mind.
This phenomenon of checking the geographical data before planning is known as geospatial analysis. It is more technical and it involves statistical data and analytics that help them set up a plan. In this article, we are going to learn in detail about what is geospatial analysis, its purpose, benefits along with its challenges and we hope this helps you to have a clear awareness of geospace from now on.
What is Geospatial Analysis?
Geospatial analysis is a type of analysis that uses data that is associated with a specific location or geographic area. This is also known as geographical data analysis which uses real-time geographic data and helps in the decision-making process.
The geospatial analysis involves gathering, displaying, manipulating, and interpreting geographical data to uncover patterns, trends, and insights that can inform decision-making processes. Geospatial analysis combines Geographic Information System (GIS) technology with statistical and spatial analysis tools to enable the exploration of spatial relationships between different data sets.
Geospatial analysis has a wide range of applications, including urban planning, environmental management, natural resource management, emergency response planning, business location planning, and many more.
It can involve analyzing data such as land use, population density, topography, weather patterns, and infrastructure, among other things. The analysis can be performed on a local, regional, or global scale and can involve various levels of complexity, depending on the nature of the data and the intended use of the analysis.
What is Geospatial Technology?
Geospatial technology refers to the use of Geographic Information Systems (GIS), remote sensing, Global Positioning Systems (GPS), and other geospatial data and tools to capture, analyze, and visualize information about the physical features and characteristics of the earth’s surface.
GIS is the foundation of geospatial technology, providing a framework for organizing, analyzing, and displaying geospatial data. Remote sensing technology, such as satellite imagery, LiDAR, and aerial photography, is used to capture high-resolution data about the Earth’s surface.
GPS technology is used to capture and store the location and movement of objects or people, while also providing a reference point for geospatial analysis.
Together, these technologies and data sources enable geospatial analysts and professionals to understand and interpret complex geographic patterns and relationships, such as land use, environmental factors, natural resources, and population demographics.
How Geospatial Analysis is Used in Real-Time?
Geospatial data analysis is used in various fields to analyze and interpret data based on its geographic location. Here are eight geospatial analysis examples of how geospatial data analytics is used in real life:
- Urban planning
Geospatial data analysis is used to analyze and understand the spatial distribution of population, infrastructure, and land use in urban areas. This information can help Urban planners make informed decisions about city planning and development.
Also Read: Urban Computing: Facing the Big Challenges of Big Cities
- Natural resource management
Geospatial data analysis is used to monitor and manage natural resources, such as forests, waterways, and fisheries. This information can help resource managers make informed decisions about the conservation and sustainable management of these resources.
- Environmental management
Geospatial data analysis is used to monitor and assess pollution levels and other environmental hazards. This information can help environmental managers make informed decisions about the remediation and prevention of environmental problems.
- Emergency response planning
Geospatial data analysis is used to map and analyze emergency response resources, such as fire stations, hospitals, and evacuation routes. This information can help emergency planners make informed decisions about emergency response planning.
- Agriculture
Geospatial data analysis is used to analyze soil quality, crop yields, and climate data. This information can help farmers make the right decisions about planting, fertilizing, and harvesting crops.
- Transportation planning
Geospatial data analysis is used to analyze traffic patterns and transportation infrastructure. This information can help transportation planners make prompt decisions about the design and construction of transportation systems.
- Business location planning
Geospatial data analysis is used to analyze customer demographics, market demand, and competitor locations. This information can help businesses make informed decisions about where to locate and how to market their products and services.
- Geology and Geophysics
Geospatial data analysis is used to map and analyze geological features, such as fault lines, mineral deposits, and underground aquifers. This information can help geologists and geophysicists make decisions about resource extraction, hazard mitigation, and other geological activities.
Overall, geospatial data analysis is a powerful tool that can be used in decision-making across a wide range of fields.
Benefits of Geospatial Analysis
There are several benefits of using geospatial data in various fields. Here are some of the key benefits:
- Better decision-making: Geospatial data can help decision-makers make more informed decisions by providing valuable insights into spatial patterns and relationships.
- Improved resource management: Geospatial data can help resource managers make more informed decisions about the management and conservation of natural resources, such as forests, waterways, and wildlife.
- Enhanced public safety: Geospatial data can help emergency responders and public safety officials make more informed decisions by providing real-time information about hazards, risks, and emergency response resources.
- Increased efficiency: Geospatial data can help businesses and organizations optimize their operations and improve their efficiency by providing insights into customer behavior, market demand, and other key factors.
- Better planning and design: Geospatial data can help urban planners, architects, and engineers make more informed decisions about the design and development of buildings, infrastructure, and cities.
- Improved accuracy and precision: Geospatial data can provide highly accurate and precise information about geographic features and relationships, which can be critical for many applications.
- Better communication: Geospatial data can be visualized and communicated in intuitive and effective ways, making it easier for stakeholders to understand and engage with the information.
Geospatial data provides a wealth of valuable information that can be used in decision-making, improve resource management, enhance public safety, increase efficiency, and support better planning and design.
Types of Geospatial Data
There are several types of geospatial data that are used in GIS (Geographic Information Systems) and other geospatial analysis applications. Here are some of the main types of geospatial data:
- Vector data: Vector data is used to represent geographic features such as points, lines, and polygons. This type of data is stored as coordinates and topological relationships between the features.
- Raster data: Raster data is used to represent geographic features as a grid of cells or pixels. Each pixel has a value that represents the characteristic of the geographic feature at that location, such as elevation or temperature.
- Satellite imagery: Satellite imagery is a type of raster data that is captured by remote sensing technologies, such as satellites or drones. This type of data is often used for land use mapping, environmental monitoring, and other applications.
- LiDAR data: LiDAR (Light Detection and Ranging) data is a type of remote sensing data that is used to create highly detailed maps of terrain and vegetation. It works by using laser pulses to measure the distance between the sensor and the ground, creating a 3D representation of the landscape.
- GPS data: GPS (Global Positioning System) data is used to capture and store the location and movement of objects or people. This type of data is often used for navigation, mapping, and tracking applications.
- Census data: Census data is used to represent the demographic and socioeconomic characteristics of a population. This type of data is often used for urban planning, marketing, and social science research.
- Weather and climate data: Weather and climate data are used to represent atmospheric conditions and climatic patterns. This type of data is often used for weather forecasting, climate modeling, and environmental monitoring.
These are just a few examples of the types of geospatial data that are commonly used in geospatial analysis. Depending on the application, different types of geospatial data may be used to represent different features and characteristics of the landscape.
Challenges and Best Practises in Geospatial Analysis
Geospatial data analysis presents a range of challenges and best practices that are important to consider in order to effectively analyze and utilize geospatial data. Here are some of the key challenges and best practices:
Challenges
- Data quality: Geospatial data can be complex and may vary in quality and accuracy. It is important to ensure that the data used for analysis is accurate, up-to-date, and relevant.
- Data integration: Geospatial data can come from a variety of sources and may need to be integrated with other types of data in order to provide useful insights. This can be a complex process that requires careful attention to detail.
- Data privacy and security: Geospatial data may contain sensitive information, such as personal data or proprietary business information. It is important to ensure data privacy and security protocols are in place to protect this information.
- Technical expertise: Geospatial data analysis requires specialized technical expertise, including knowledge of GIS software, remote sensing technologies, and statistical analysis techniques.
- Interpretation and visualization: Geospatial data can be complex and difficult to interpret and visualize. It is important to ensure that the results of the geospatial analysis are presented in a way that is clear and understandable to stakeholders.
- Infrastructure and resources: Geospatial data analysis can require significant infrastructure and resources, including high-performance computing, data storage, and network bandwidth.
Best Practices
- Data management: Effective geospatial data analysis requires careful attention to data management practices, including data cleaning, quality control, and documentation.
- Collaboration: Collaboration with experts from different fields can help ensure that geospatial data analysis is informed by a wide range of perspectives and expertise.
- Clear objectives: Geospatial data analysis should be guided by clear objectives and research questions in order to ensure that the analysis is relevant and useful.
- Continuous learning: Geospatial data analysis is a rapidly evolving field, and it is important to stay up-to-date with the latest tools, techniques, and best practices.
- Visualization and communication: Effective visualization and communication of geospatial data analysis results can ensure that stakeholders understand the implications of the analysis and can make informed decisions based on the results.
- Quality control and assurance: Geospatial data analysis should include a rigorous quality control and assurance process to ensure that the results are accurate and reliable.
- Standardization and interoperability: Geospatial data analysis should be based on standard formats and protocols to ensure that the results can be shared and used by others. Interoperability between different data sources and analysis tools is also essential to ensure that data can be effectively integrated and analyzed.
Geospatial data analysis presents a range of challenges and opportunities. By following best practices and addressing challenges such as data quality, integration, privacy, and technical expertise, organizations and individuals can leverage the power of geospatial data analysis to understand better and manage the complex geographic patterns and relationships that shape our world.
FAQ on Geospatial Data Analysis
1. What is geospatial analysis?
Geospatial analysis is the process of analyzing, modeling, and visualizing data that has a geographic or spatial component. This type of analysis allows researchers to identify patterns, trends, and relationships in data that are related to geographic location.
2. What types of data can be used in geospatial analysis?
Geospatial analysis can use a variety of data types, including satellite imagery, aerial photography, GIS data, and other types of spatial data. This data can be used to analyze anything from weather patterns to population demographics.
3. What are some applications of geospatial analysis?
Geospatial analysis has many applications, including urban planning, disaster response, environmental management, and public health. It can also be used in business, marketing, and other fields to help organizations make informed decisions based on spatial data.
4. What software is commonly used for geospatial analysis?
There are many software options available for geospatial analysis, including proprietary software like ArcGIS and QGIS, as well as open-source tools like R and Python.
5. What are some challenges in geospatial analysis?
Some challenges in geospatial analysis include data quality and integration, data privacy and security, technical expertise, and interpretation and visualization. Additionally, geospatial data analysis can require significant infrastructure and resources.
6. What are some best practices for geospatial analysis?
Best practices for geospatial analysis include effective data management, collaboration, clear objectives, continuous learning, visualization and communication, quality control and assurance, and standardization and interoperability.
7. What careers involve geospatial analysis?
There are many careers that involve geospatial analysis, including geospatial data analyst, cartographer, surveyor, urban planner, environmental scientist, and public health analyst. Additionally, many businesses and organizations use geospatial analysis to inform decision-making and strategy.
High impact and greater visibility for your work
Infographics help bring high impact and greater visibility for your work as a scientist. You can unlock the potential of infographics with Mind the Graph. Sign up now to explore a huge library of visual abstracts that align with your research paper.
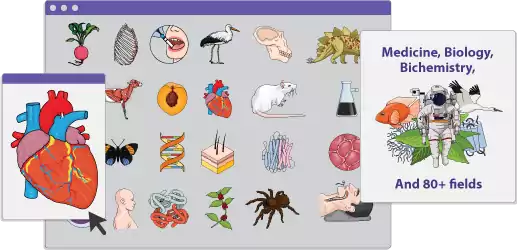
Subscribe to our newsletter
Exclusive high quality content about effective visual
communication in science.