When it comes to scientific research, figuring out why things happen is essential. This is where experimental design becomes indispensable, helping researchers uncover the secrets behind cause-and-effect relationships. By carefully planning experiments, collecting precise data, and analyzing them thoughtfully, experimental design gives researchers the tools to identify and understand how different things are connected. In this article, we’ll explore the field of experimental design, understand its importance, purpose, and the different methods used in various areas of study.
What Is Experimental Design?
Experimental design is a systematic approach to planning, conducting, and analyzing experiments to identify and understand causal relationships between variables. It involves carefully designing the experiment to control for potential confounding factors and to ensure that data collection efforts effectively address research questions and hypotheses. Experimental design encompasses the selection of factors and treatments, the assignment of participants or subjects to different conditions, and the collection and analysis of data to draw meaningful conclusions. By employing various experimental designs, such as completely randomized, randomized block, and observational Studies, researchers can enhance the validity and reliability of their findings.
Types Of Experimental Design
Experimental designs encompass various types that can be customized to fit specific research objectives and settings. Each design offers unique advantages and limitations, allowing researchers to control for confounding factors, examine interaction effects, or work within ethical constraints. Here are some common types of experimental designs:
Independent Measures
Independent Measures, also known as between-subjects design or independent groups design, is a concept in experimental design where different participants are assigned to different experimental conditions or groups. In this design, each participant experiences only one level of the independent variable, and their responses or outcomes are compared across these different groups.
Using independent measures allows researchers to simultaneously examine multiple conditions, reducing the potential influence of individual differences and participant-related variables. However, it requires a larger sample size and poses the risk of unequal group compositions. To address this, random assignment is often employed to ensure that participants are distributed randomly across the different conditions.
Matched Pairs
In experimental design, researchers have various options to address the challenge of reducing variability and obtaining reliable results. One approach is the use of a matched pairs experimental design, which falls under the category of between-subjects studies. In this design, researchers aim to minimize pre-existing differences between experimental groups by pairing subjects with similar characteristics. Each pair consists of two participants, with one assigned to the treatment group and the other to the control group.
While it may not achieve the same level of matching as within-subjects designs, matched pairs design helps reduce variability between groups and avoids treatment order effects. However, this approach can be time-consuming and is dependent on finding suitable matched pairs. Overall, selecting an appropriate experimental design requires careful consideration of subject area concerns, available resources, and the research question at hand.
Repeated Measures Design
Repeated Measures Design, also known as within-subjects design, is an experimental approach where the same participants are exposed to multiple conditions or levels of an independent variable. Measuring participants’ responses across conditions allows for the examination of within-subject differences while minimizing individual variability. However, it is important to address potential order effects through counterbalancing techniques.
Repeated measures design offers advantages such as increased statistical power and insights into individual variations. Analyzing the data often involves specialized statistical techniques. Overall, repeated measures design provides a valuable method to study changes within participants and understand the effects of independent variables while controlling for individual differences.
Between-subjects Vs. Within-subjects
In a between-subjects design, participants are assigned to different groups, such as a treatment group or a control group, and the groups are compared at the end of the experiment. This approach, also known as independent measures design, ensures that each participant is exposed to only one condition. However, pre-existing differences between groups can potentially impact the results, despite efforts to randomize assignments.
On the other hand, a within-subjects design, also referred to as a repeated measures design, involves participants experiencing all treatment conditions and being measured for each one. This design allows each participant to serve as their control, reducing variability and increasing statistical power. However, the order in which treatments are administered can influence the results, and researchers need to be mindful of potential practice and fatigue effects.
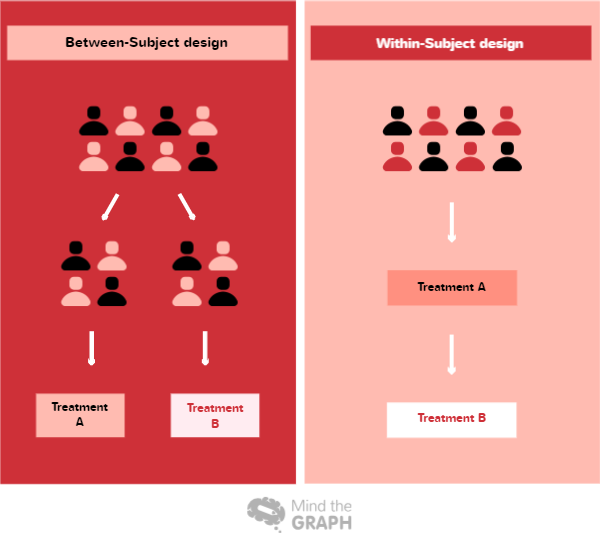
Choosing between a between-subjects design and a within-subjects design requires careful consideration of the research objectives, the nature of the studied variables, and potential confounding factors.
Developing An Experimental Design
Developing an experimental design involves careful planning to optimize the collection of trustworthy data and detect causal relationships. The primary objective of these studies is to observe effects that exist within the population under investigation, with a preference for identifying causal effects. This requires isolating the true effect of each factor from potential confounding variables and generating conclusions that can be generalized to the real world.
To achieve these goals, experimental designs prioritize data validity and reliability and internal and external experimental validity. When an experiment is valid and reliable, researchers can have confidence in the accuracy and consistency of their procedures and data, leading to reliable results.
A successful experimental design involves the following key components:
Preplanning
Significant emphasis is placed on thorough preplanning, where researchers carefully consider the research question, the variables of interest, and the overall design of the experiment. This ensures that all necessary aspects are taken into account before the study begins.
Developing Experimental Treatments
Researchers design and define the treatments or conditions that participants will experience during the experiment. These treatments are carefully designed to manipulate the variables of interest, allowing researchers to assess their effects.
Determining Subject Assignment To Treatment Groups
Researchers must decide how to assign participants or subjects to different treatment groups. This can be done randomly or through other systematic methods to ensure fairness and minimize potential biases.
Assigning Subjects To Experimental Groups
The assignment of subjects to experimental groups is a critical aspect of experimental design. Researchers must carefully determine how participants are allocated to the treatment and control groups. The control group typically represents the absence of treatment and provides a baseline for comparison. The method of assigning subjects to groups significantly impacts the ability to establish true causal effects and control for confounding variables. Let’s examine some approaches to allocating subjects within experimental designs.
Completely Randomized
Completely randomized designs involve randomly assigning subjects to treatment and control groups using methods like flipping coins, rolling dice, or using a computer. This random assignment ensures the groups are roughly equivalent at the beginning, increasing confidence in attributing any differences observed at the end to the treatments rather than other factors. Randomization helps equalize confounders between the groups, leaving only the treatment effects.
An example of a completely randomized design is a study investigating the effects of a new teaching method on student performance. Researchers randomly assign students to two groups: one group receives the new teaching method, while the other group continues with the traditional teaching method. If there are noticeable changes in outcomes by the end of the study, researchers can be sure that the improvements were caused by the new method.
Randomized Block
Randomized block designs are employed when nuisance factors, which can impact outcomes but are not the primary focus of the research, are present. These factors have the potential to conceal or distort treatment effects. To mitigate their influence, experimenters use a randomized block design.
In this design, subjects sharing a common nuisance characteristic are organized into blocks, and participants within each block are randomly assigned to the experimental groups. This approach allows for controlling known nuisance factors. By incorporating blocking in experimental designs, the impact of nuisance variables on experimental error is reduced. The analysis examines treatment effects within each block, removing variability between blocks. Consequently, blocked designs enhance the accuracy of detecting treatment effects by minimizing the influence of nuisance variables.
For instance, if testing different teaching methods, grade level may be a relevant nuisance factor influencing educational outcomes. To implement a randomized block design, participants would be divided by grade level, and members within each level would be randomly assigned to the experimental groups.
Observational Studies
Observational studies, also known as quasi-experimental designs, are utilized in situations where it is impractical or unethical to randomly assign subjects to experimental conditions. Instead, researchers observe subjects within their natural groupings, measure critical variables, and search for correlations.
Observational studies allow for research when treatment control is not feasible. However, quasi-experimental designs introduce challenges related to confounding variables. In this type of experimental design, the correlation between variables does not necessarily indicate causation. While specific procedures can help manage confounders in observational studies, confidence in establishing causal findings is ultimately lower.
For example, imagine you’re studying the impact of exercise on weight loss. It’s not possible to randomly assign participants to exercise and non-exercise groups. However, you can compare individuals who engage in regular exercise with those who don’t and observe how their weight loss outcomes vary.
Treatments In Experimental Designs
In experimental design, treatments refer to the variables that researchers manipulate and control during the study. These treatments serve as the primary independent variables of interest, and the researchers administer them to the subjects or items involved in the experiment. The purpose is to observe whether the treatments have an impact on the outcome or dependent variable.
While treatments can include medical interventions like medications or vaccines, the term extends to various other factors such as training programs, teaching methods, manufacturing settings, or types of fertilizers. When determining the treatments, it is crucial to carefully consider their specific characteristics, such as the dosage or intensity. For example, if comparing three different temperatures in a manufacturing process, it is necessary to define the specific variations between them.
The way treatments are defined and designed within the experiment can significantly impact the results obtained and the generalizability of the findings. Therefore, careful consideration and precise specification of the treatments are important aspects of experimental design to ensure accurate and meaningful conclusions.
Visually Appealing Figures For Your Research
Mind the Graph offers scientists the ability to create visually appealing figures for their research. With a user-friendly interface and an extensive library of scientific illustrations, researchers can easily customize templates, charts, and graphs to convey their findings engagingly. The platform also provides access to scientifically accurate illustrations, allowing researchers to visually represent complex concepts and structures. By enhancing the visual impact of their research through visually appealing figures, scientists can effectively communicate their work and captivate their audience.
Subscribe to our newsletter
Exclusive high quality content about effective visual
communication in science.